Українською
  In English
ELE Times


STMicroelectronics Publishes 2024 Sustainability Report
- On track to become carbon neutral on scope 1 and 2, and partially scope 3, by 2027 with global sourcing of electricity from renewable energy growing to 71% in 2023, from 62% in 2022
- 87% of employees would recommend ST as a great place to work
- 12.2% of net revenues (US$2.1 billion) invested in R&D to support innovation
STMicroelectronics, a global semiconductor leader serving customers across the spectrum of electronics applications, today releases its annual sustainability report, which provides insight into its 2023 performance related to priority environmental, social and governance (ESG) factors that support long-term value creation for all its stakeholders and sustainable growth for its business.
“Sustainability is at the heart of our value proposition, driving benefits for our company, customers, and society. We’re proud of our progress on innovation, resilience, employee engagement and the advancement towards our ambitious goal of becoming carbon neutral on scope 1 and 2, and partially on scope 3, by 2027,” said Jean-Marc Chery, President and CEO, STMicroelectronics. “Together with our partners and empowered employees, we’re accelerating sustainable transformation in technology and beyond.”
ESG highlights during 2023 include:
- A 45% decrease in GHG emissions for scopes 1 and 2 since 2018 in absolute terms (vs a 40% decrease in 2022).
- An increased sourcing of electricity coming from renewable sources to 71% (from 62% in 2022) and the signing of a significant power purchase agreement (PPA) in Italy to produce 250GWh renewable energy per year (3.75 TWh over 15 years) starting in 2024.
- The reuse, recovery, or recycling of 96% of the Company’s waste (1% higher than in 2022).
- A- scores for CDP water security and climate change.
- 12.2% of net revenues (US$2.1 billion) invested in R&D to support innovation.
- Involved in 195 active R&D partnerships worldwide.
- More than 610 STEM (Science, Technology, Engineering, and Mathematics) events and initiatives (a 35% increase on 2022) reaching over 100,000 students and teachers globally.
- 87% of employees would recommend ST as a great place to work, 4% higher than in 2021 (when we last had a full survey).
- In 2023, the company established a strong focus on setting up voluntary, employee-led Employee Resource Groups (ERGs) to promote inclusion in the workplace. WISE (Women Inspiring Supporting and Empowering), the first ERG for women, has grown fast, with some 1,400+ members in 60 locations.
In 2023, ST maintained a strong presence in major sustainability indices such as the Dow Jones Sustainability indices, FTSE4Good, EuroNext VIGEO Europe 120, CAC 40 ESG, MIB ESG, ISS ESG Corporate ratings, Bloomberg Gender Equality Index, and received an MSCI ESG Rating of AAA.
The 27th annual report contains highlights and details of ST’s sustainability performance in 2023 and presents the Company’s ambitions and longer-term goals in alignment with both the United Nations Global Compact Ten Principles and Sustainable Development Goals and the Science Based Targets initiative (SBTi). It is aligned with Global Reporting Standards (GRI), Sustainability Accounting Standards Boards (SASB), and Task Force on Climate-Related Financial Disclosures (TCFD). A third party has verified this report.
The post STMicroelectronics Publishes 2024 Sustainability Report appeared first on ELE Times.
STMicroelectronics reveals advanced ultra-low-power STM32 microcontrollers for industrial, medical, smart-metering, and consumer applications
New flagship in ultra-low-power consumes less, and delivers more at an effective cost
STMicroelectronics, a global semiconductor leader serving customers across the spectrum of electronics applications, has introduced a new generation of energy-conscious and cost-effective microcontrollers (MCUs) that can reduce energy consumption by up to 50% compared to previous product generations. This enables less frequent battery replacements, minimizes the impact of discarded batteries, and allows more designs to go battery-freerunning solely from an energy-harvesting system such as a small photovoltaic cell.
In the global pursuit of sustainability, technologies deployed in smart buildings and Internet of Things (IoT) applications are critical tools for managing energy and resources efficiently. At the heart of the smart sensors and actuators making them possible, ST’s microcontrollers manage the processes that collect, filter, analyze, and act, communicating with high-level applications in the cloud. There are billions of these MCUs in action already and the expansion of smart living and working will demand billions more.
“It’s vital that these pervasive devices consume as little energy as possible as they help to minimize waste elsewhere. The new STM32U0 microcontroller series we are introducing today takes this notion to a new level, building on our proven ultra-low-power technologies,” explained Patrick Aidoune, General Purpose MCU Division General Manager, STMicroelectronics. “In fact, our new MCUs consume so little power that small devices in some dedicated applications like industrial sensor management can run for twice as long from the same size battery. This comes with the opportunity to add more advanced features and deliver cost-effective solutions for a variety of industrial, medical and consumer devices.”
The new STM32U0 MCUs enable this great leap in energy efficiency through a combination of their state-of-the-art design techniques and advanced manufacturing process. These include extremely low static power consumption in standby mode and superior wake-up performance, enabling the MCU to spend more time in power-saving sleep modes to minimize average energy demand.
One lead customer, in the security-systems market, is using the STM32U0 in security cameras to wake the device when motion is detected and thus enhance surveillance while saving energy. Another has created ultra-long-lasting smoke detectors, while a further application, by Ascoel, is using the STM32U0 to manage power-conscious functions of a water meter.
“Ascoel builds a wide range of innovative electronic devices with a focus on IoT technologies. We are working to design an ultrasonic water meter, with battery lasting for more than 10 years, integrating an LCD screen for real-time data, as well as the necessary security,” said Francesco Cerretani, Senior Software Developer, Ascoel. “We found that the STM32U0 was the perfect solution, supporting our goal to create a low-cost, ultra-low-power, easy-to-install, zero maintenance, precise water meter. When deployed at scale in the customer’s grid, it will improve control over water leakage and contribute to reducing the environmental impact.”
The STM32U0 enhances cost-effectiveness by providing an LCD segment display controller. Devices with an LCD, like Ascoel’s water meter as well as thermostats, smart retail labels, access-control panels, and factory automation can take advantage of this to reduce the cost of their PCB. Additional value-added features of STM32U0 MCUs include numerous analog peripherals like analog-to-digital converters (ADC), digital-to-analog converters (DAC), operational amplifiers, and comparators. There is also an on-chip system oscillator that helps cut the bill of materials to save costs and PCB space.
STM32U0 devices are the first MCUs running on Arm Cortex-M0+ targeting SESIP Level 3 and PSA level 1 focusing on firmware code protection. Certification provides an independent assurance of the STM32U0 security capabilities that product manufacturers can leverage to comply with the coming voluntary US Cyber Trust Mark and mandatory EU Radio Equipment Directive (RED).
Developers can also take advantage of up to 256KB of Flash, package options up to 81 pins, and 56MHz core speed, which are generous specifications for this class of device.
The STM32U0 series is in production now and ST is making the new devices available at an attractive price point starting at $0.68 for 1,000 units. Sample requests and further pricing options are available from local ST sales offices.
For more information, please go to www.st.com/stm32u0.
A marketing presentation for the STM32U0 series is available at https://www.st.com/resource/en/product_presentation/microcontrollers-stm32u0-series-product-overview.pdf.
STM32 is a registered and/or unregistered trademark of STMicroelectronics International NV or its affiliates in the EU and/or elsewhere. In particular, STM32 is registered in the US Patent and Trademark Office.
The post STMicroelectronics reveals advanced ultra-low-power STM32 microcontrollers for industrial, medical, smart-metering, and consumer applications appeared first on ELE Times.
How Intelligent Power Modules Are Making Heat Pumps Smarter
The demand for more efficient power semiconductors is on the rise as businesses adapt to a low-carbon future. Minimizing the overall cost and footprint of the system while enhancing efficiency is a crucial objective that needs to be considered while developing power semiconductor solutions. Intelligent power modules (IPMs) are one such solution that has gained significant attention in the heat pump market. These compact and highly integrated modules provide high power density and advanced control and monitoring capabilities, making them an ideal choice for heat pumps.
The Importance of Heat PumpsAccording to Eurostat data, about 50% of all energy consumed in the European Union (EU) is used for heating and cooling, and more than 70% still comes from fossil fuels (mostly natural gas). In the residential sector, around 80% of the final energy consumption is used for space and water heating.
Heat pumps (Figure 1) can deliver heating, cooling, and dehumidification needs and are a proven and sustainable solution for heating powered by electricity. They are an environmentally friendly option for your home and office, helping us move away from fossil fuels to more renewable energy technologies. With global concerns about energy security and climate commitments, heat pumps are emerging as the primary tool for decarbonization in space and water heating systems.
The number of heat pumps installations globally will rise from 180 million in 2020 to around 600 million in 2030, according to the International Energy Association (IEA). At least three times more efficient than traditional fossil fuel boilers, heat pumps are preferred and its installation in individual buildings is forecasted to rise from 1.5 million per month currently to around 5 million by 2030 (Source: IEA). In the U.S., the Inflation Reduction Act includes tax credits and rebates that can cover up to 100% of the costs, depending on household eligibility.
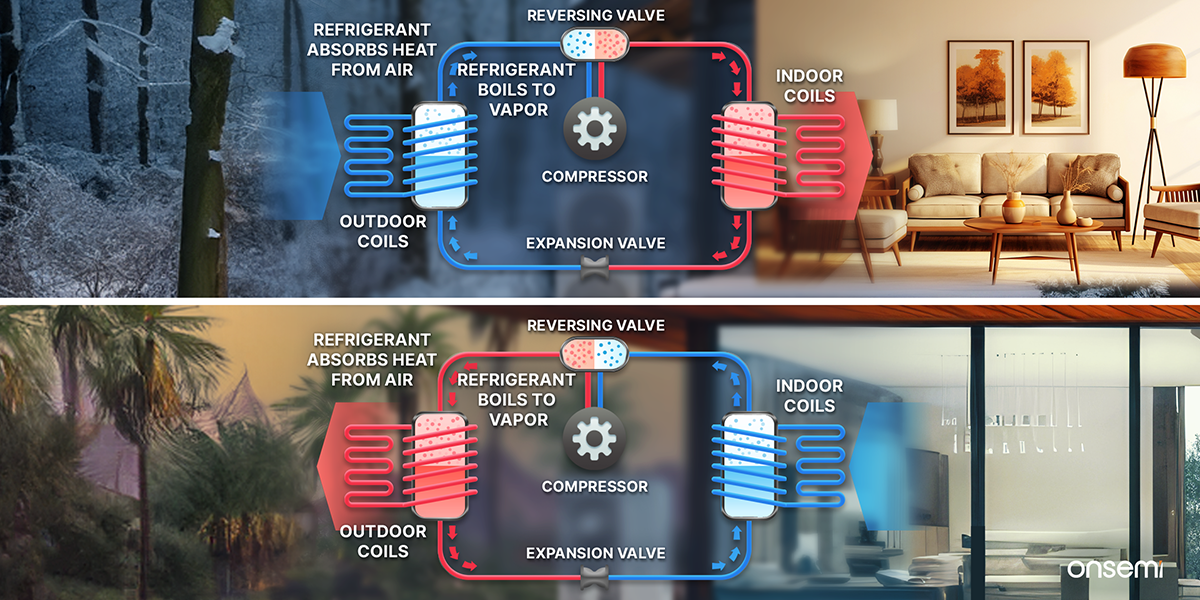
Intelligent power modules (IPMs) are crucial in controlling the power flow to inverter compressors and fans in heat pump systems. (Figure 2) These modules adjust the frequency and voltage of power supplied to three-phase motors and contribute to achieving higher energy efficiency standards for compressors and pumps. For example, coolers using IPMs for their inverter systems reduce power by 30% compared to non-inverter systems.
IPMs are prevalent in various applications and systems. Owing to their high level of integration, whether in the power stage, drivers, or protections, IPMs allow for shorter development phases.
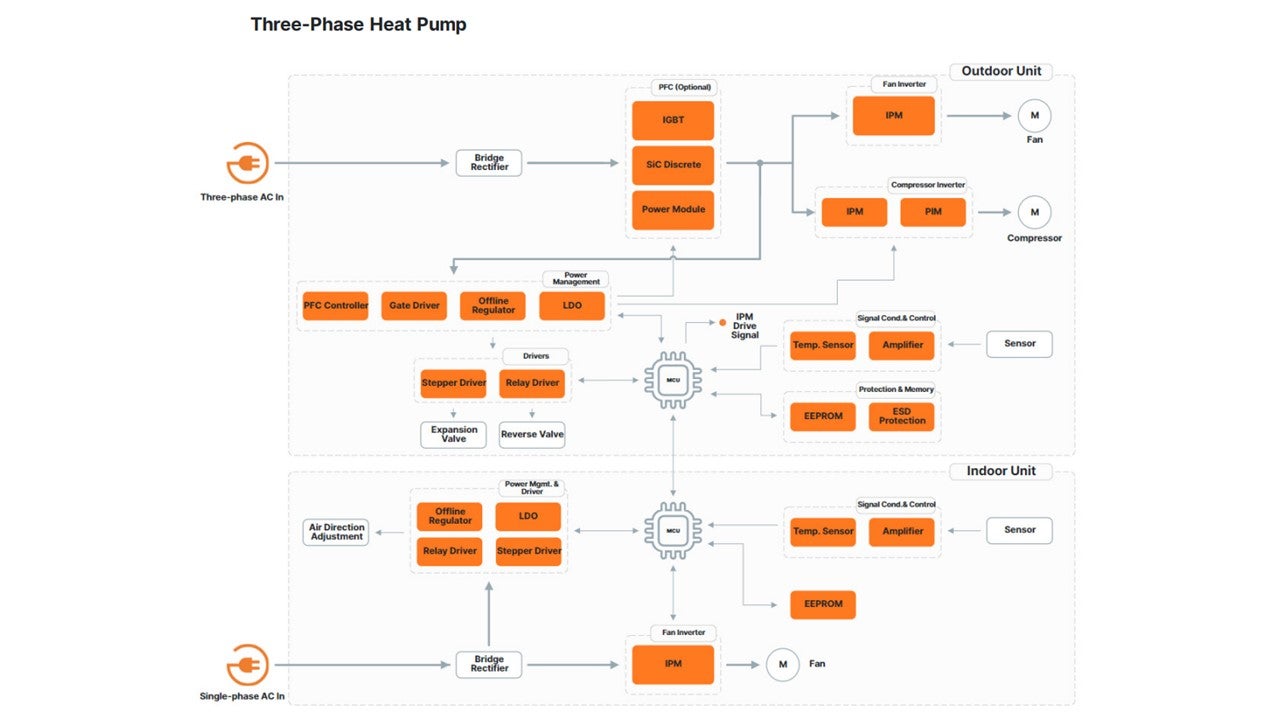
onsemi has recently unveiled a new family of 1200 V SPM31 IPMs, setting a new standard for three-phase inverter applications. This groundbreaking technology not only addresses the growing need for energy efficiency but also focuses on reducing system costs and improving overall performance. With new and improved features, the SPM31 IPMs are a game-changer in the heat pump market.
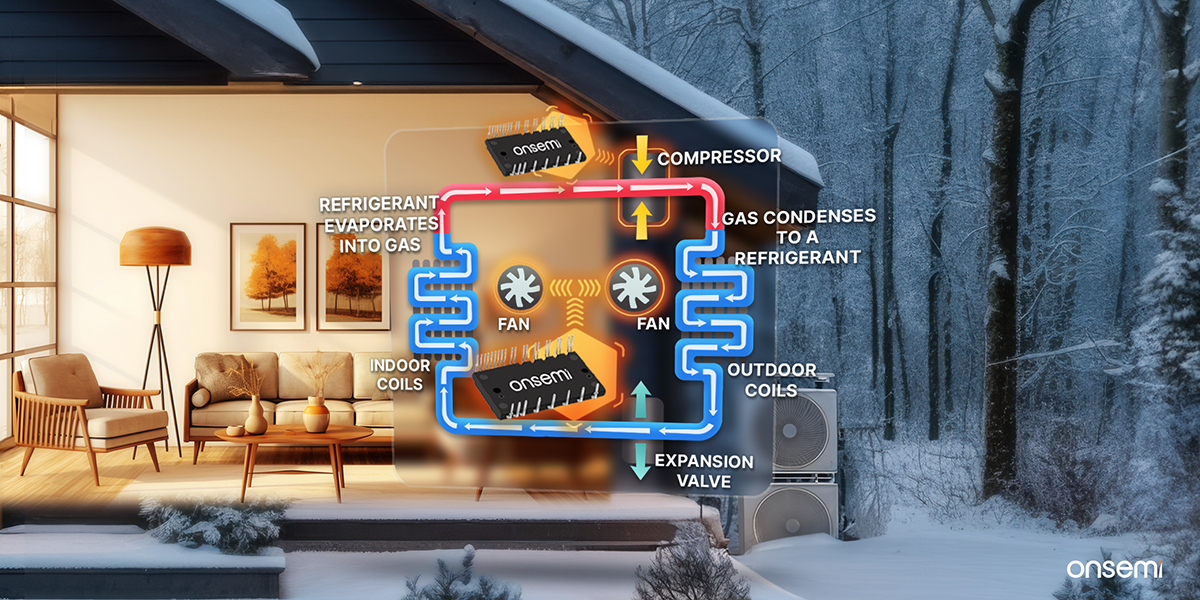
The SPM31 IPMs incorporate the latest 7th generation of IGBT Field Stop (FS7) technology, making them highly efficient and robust. The well-optimized active cell design and buffer profile, coupled with narrow electrical parameter distribution, eliminates short circuit oscillation in both single and parallel device operations. This technology uses a submicron trench gate cell pitch that increases channel density to reduce the conduction losses. The gate capacitance is optimized for smooth switching waveforms and low switching losses. On the emitter side, multiple FS layers enhance blocking capability and reduce drift layer thickness, resulting in lower conduction and switching energy losses. The FS7 IGBT development focuses on optimizing both Vcesat and Eoff to achieve state-of-the-art device performance.
This technology results in minimized EMI, up to 10% a remarkable reduction in power losses, up to 9% increase in power density, and a 20% size reduction in IGBT chip die size compared to previous generation products. As a result of the improved power density, designers can use a simplified layout to free up valuable space in the heat pump system while improving efficiency. The SPM31 IPMs are available in multiple current rating line-ups ranging from 15 to 35 Amps (A).
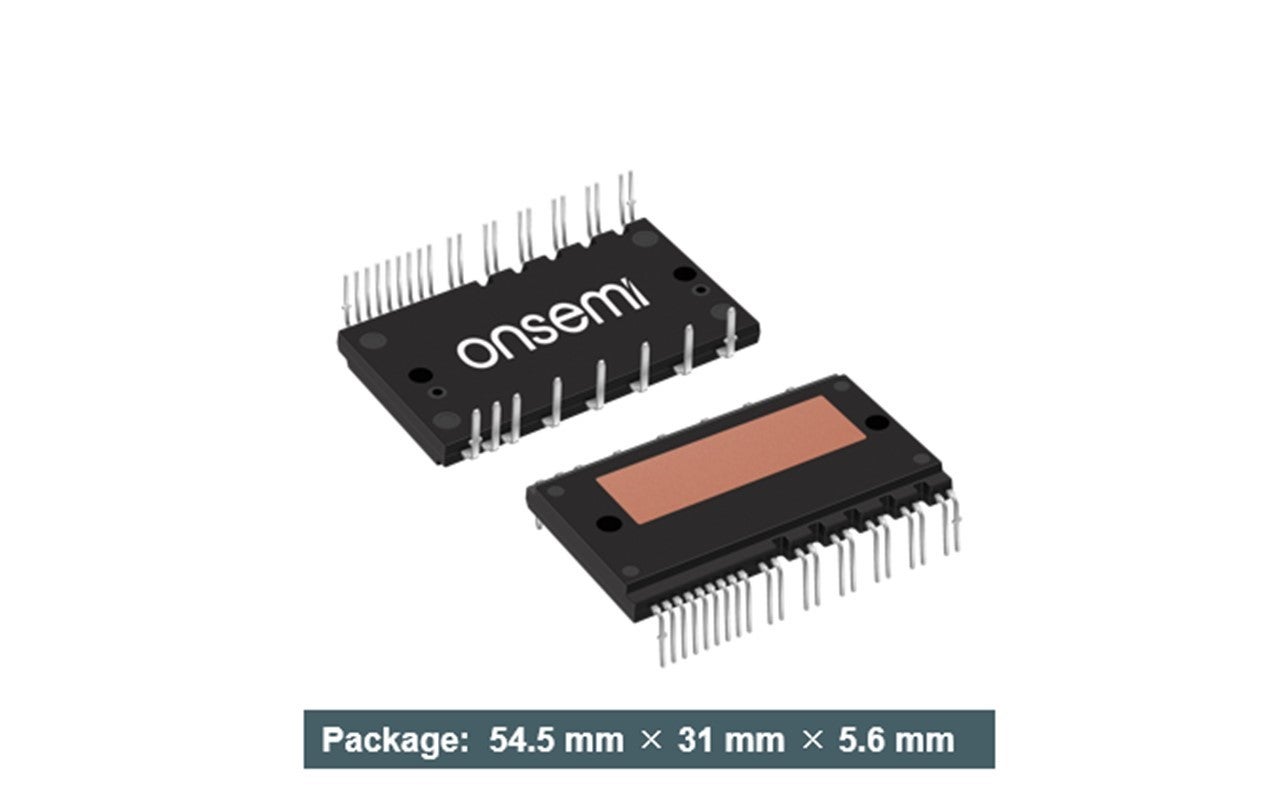
onsemi’s SPM31 IPMs come equipped with an array of advanced features to ensure reliable operation. The modules include gate driver ICs and various on-module protection features, such as:
- Under-Voltage Lockouts: The SPM31 IPMs protect against low voltage conditions that can cause unpredictable operation or even damage to the system.
- Over-Current Shutdown: This feature provides protection against excessive current flow, which can cause permanent damage to the system.
- Thermal Monitoring: The SPM31 IPMs can accurately sense temperature, allowing for proper thermal management and preventing overheating.
- Fault Reporting: The modules can detect and report various faults to the system, providing early warning signs for potential issues.
Additionally, the SPM31 IPMs use a direct bond copper substrate (Figure 5), providing superior thermal performance. These features enhance the robustness of the modules and contribute to their suitability for a wide range of applications. The versatility of the SPM31 IPMs makes them an ideal choice for various inverter drive applications including HVAC, heat pumps, variable frequency drives (VFD), industrial pumps and fans, and servo motors.
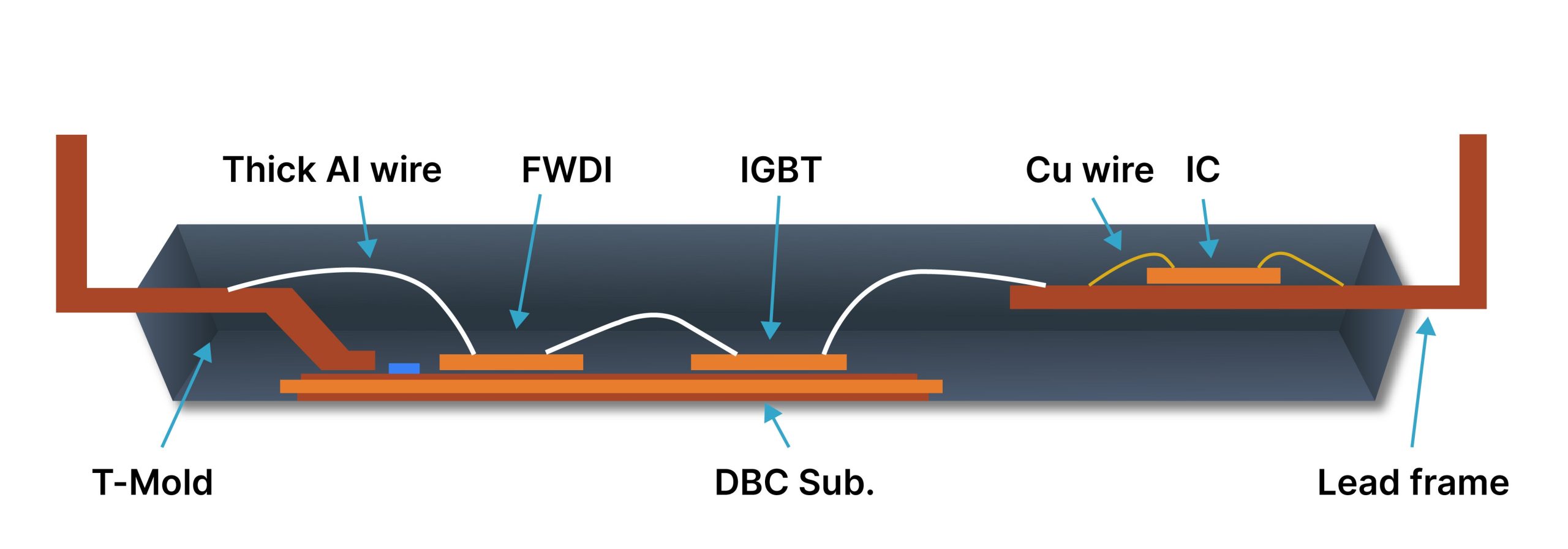
Alongside the SPM31, onsemi has a versatile IPM product portfolio. (Figure 6)
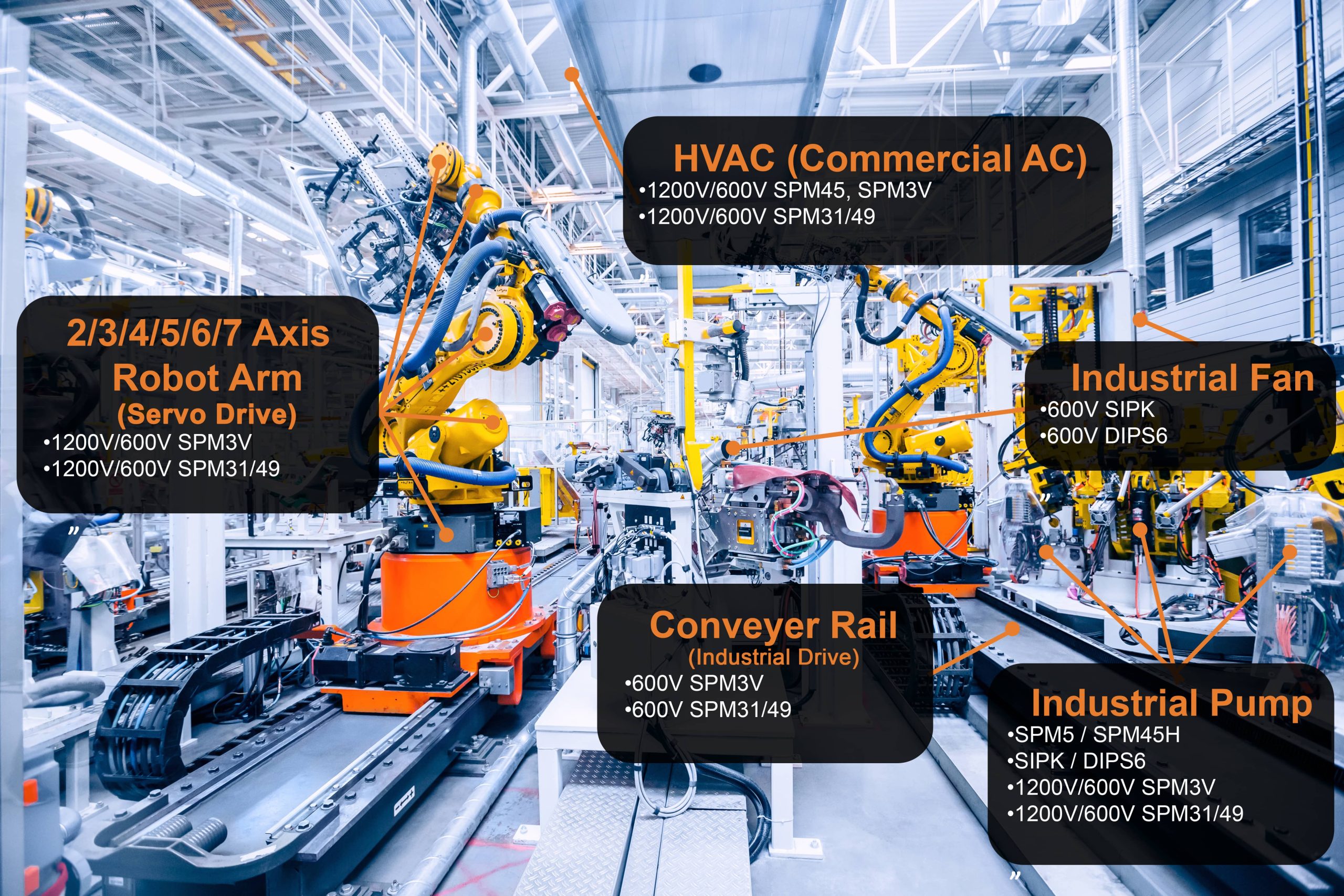
The adaptability of these modules showcases their potential to revolutionize multiple industries by providing efficient and compact solutions.
A Leap Forward for Heat Pump ApplicationsThe SPM31 IPMs are a significant advancement for heat pumps. The IPMs provide a compact, high-performance solution that helps engineers design more efficient, reliable, and cost-efficient systems for industrial applications.
The post How Intelligent Power Modules Are Making Heat Pumps Smarter appeared first on ELE Times.
How to Select a Timer on PIC MCUs
Timers are a common peripheral in microcontrollers (MCUs), including PIC MCUs. With so many possible choices, how do you select the right one to use in your application?
Exploring the Versatile World of Microcontroller (MCU) Timers: Applications and Selection GuideTimers are a ubiquitous peripheral in MCUs. It should come as no surprise that there are a lot of timers, each with their own use case that they excel at. Some timers are designed to be used as part of waveform generation, while others are ideal for pulse counting. In many cases, there is no wrong choice—instead, it will depend on the requirements and resources available. For more information about the timers, please see the linked peripheral pages in the table below.
*Hardware limit refers to the ability to rollover at an arbitrary value, rather than the maximum count possible. (E.g.: 0x1000 versus 0xFFFF for a 16-bit timer)
TMR0 can function in 8-bit or 16-bit mode. When in 8-bit mode, the high and low byte of the timer are independent of each other. The timer rolls over when the value set in the high byte matches the low byte. 16-bit mode is a free running timer, where the timer will rollover when it reaches the value 0xFFFF. To prevent data corruption during read/writes, the register is buffered, and only latched on the low byte.
Timer 1/3/5/… (TMR1)TMR1 is a 16-bit gated timer with support for both synchronous and asynchronous clock signals. When TMR1 is in asynchronous mode, the timer functions in sleep and can generate an interrupt to wake the microcontroller. The timer also contains a gating function which can be used to hold the current value. To prevent corruption when reading/writing the 16-bit value, the timer can be configured to buffer the counter. The data will be latched on the low byte.
Timer 2/4/6/… (TMR2)TMR2 is an 8-bit timer that supports one-shot and monostable modes of operation. One-shot mode triggers the timer, then clears the ON bit after reaching the hardware limit. Monostable functions identically to the one-shot mode, except that the ON bit remains set and the timer can be retriggered. TMR2 can be reset or triggered by an external signal.
Signal Measurement Timer (SMT)The SMT is a large 24-bit timer that supports the following modes:
- (Windowed/Gated) Counter
- Capture
- Time of Flight
- (Gated) Window Measurement
- High and Low Measurement
- Period and Duty Cycle Measurement
- (Gated) Timer
Note: Values in parentheses are other modes available, e.g.: Windowed Counter, Gated Counter and Counter are all valid modes.
To support these operating modes, the SMT contains four 24-bit registers. The exact behavior of the registers depends on the mode.
Universal Timer (UTMR)The UTMR is composed of two timer modules that can operate independently of each other, or as one larger timer. The size of the UTMR may vary by device; currently on the PIC18-Q71 family, it is 16-bit per module, or 32-bits if chained together. This timer was designed to contain the functionalities of all legacy timers (TMR0, TMR1 and TMR2).
The UTMR supports both synchronous and non-synchronous clock sources and allows for reading the current count without stopping the timer, even with non-synchronous sources. To control the timer, there are three configurable events: Start, Reset and Stop.
Start events define what starts the timer. The reset event defines what resets the count back to zero. And there is a stop event, which defines what will stop the timer completely. These events can be always enabled, triggered from an input signal or disabled entirely. This enables features like monostable triggering, hardware limits and one-shot operation.
Numerically Controlled Oscillator (NCO)Note: The size of the NCO (16-bits or 20-bits) may vary, depending on device family.
The NCO is designed to generate a periodic waveform by adding a programmable increment to an accumulated total. When the total overflows, the overflow is kept, and a pulse is generated. The pulse can be a fixed number of input clock cycles in width, or it can be 50% output at the cost of halving the output frequency. While the NCO isn’t designed for measurement, it can be used if the NCO is stopped, read and then restarted.
Capture/Compare/PWM (CCP)The CCP has three modes—Capture, Compare or Pulse Width Modulation (PWM). The Capture/Compare modes utilize TMR1 while PWM utilizes TMR2.
The Capture mode stores the value in TMR1 when a rising or falling event occurs (depending on operating mode). The Compare mode generates an output when the value in TMR1 matches the set value in the CCP. For PWM mode, TMR2 is 8-bit, but the CCP extends it to 10-bit using the internal oscillator’s prescaler bits.
PWMNote: For PWM that depends on TMR2, see the CCP section.
The 16-bit PWM peripheral is fully standalone—meaning that it does not utilize another system timer, unlike CCP (which depends on TMR2). There are five operating modes for generating PWM:
- Left Aligned
- Right Aligned
- Center-Aligned
- Variable Aligned
- Compare
These modes change how the count is used to generate the output. Inside of each instance are slices, each containing two outputs. The outputs share a common frequency but have their own duty cycle registers. Additionally, the peripheral is double buffered for smooth output changes and can be synchronized with other PWM instances.
Watchdog Timer (WDT)/Windowed Watchdog Timer (WWDT)The WWDT is a special timer designed to detect a deadlock in the microcontroller. A WWDT is a timer that runs the background. Periodically, software must clear it through a special sequence, or it will reset the microcontroller. WDT and WWDT differ only in that the WWDT has a “Window” feature. The “Window” feature can require the clearing sequence to be performed within a certain time window, rather than any time before the timer rolls over. This prevents a deadlock from being undetected by clearing the timer continuously.
Selecting the Timer and Learning MoreAfter understanding the timer peripherals, select which timer closest matches the features needed in the application. In many cases, there are multiple possible timers that can perform the task. In this case, select the simplest timer—this leaves timers with more capabilities available for future use. The device datasheet, application notes and technical briefs go into more detail on how all of these timers operate and the registers associated with them. Code examples that use a specific timer can be found by searching MPLAB Discover.
The post How to Select a Timer on PIC MCUs appeared first on ELE Times.
Infineon receives “GaN Strategic Partner of the Year” award from Chicony Power Technology
Chicony Power Technology, a worldwide leading manufacturer of power supplies and a pioneer in power electronics, has announced the winners of its Annual Partner Awards, honoring Infineon Technologies AG as its 2023 “GaN Strategic Partner of the Year”.
Infineon has been recognized by Chicony Power as its top partner for gallium nitride (GaN)-based power supplies, including notebook adapters, as well as ICT applications in gaming, storage and servers. This acknowledgment is the result of Infineon’s high standards for product selection, application expertise, high reliability and cost-effectiveness.
GaN stands out as one of the most crucial technologies which are essential for improving the efficiency of power supplies and reducing their product size. Pooling Infineon’s leading GaN expertise and Chicony Power’s remarkable capabilities in power supply system design, the win-win collaboration has helped push the boundaries of innovation and further strengthened both companies’ leading positions in energy-efficient power solutions. As of today, the GaN adoption rate in Chicony Power’s high-watt adapters has reached 20 percent, and this rate is rapidly increasing.
“Unrivalled R&D resources, a comprehensive application understanding and a large number of customer projects let Infineon continuously drive its roadmap for becoming a leading GaN Powerhouse,” said Adam White, Division President Power & Sensor Systems at Infineon Technologies. “The Strategic Partner of the Year award from Chicony Power is a great honor for us. We see this as part of our common mission to drive decarbonization and digitalization together.”
“We’re pleased to honor Infineon, which has played a pivotal role in driving customer success throughout 2023, as our GaN Strategic Partner of the Year,” said Peter Tseng, President of Chicony Power Technology. “Our Vision is to be a global pioneer in the implementation of new technology that enhances power supply efficiency, reduces the carbon footprint of power supplies and helps create a greener world. We would like our Annual Partner Awards to encourage Infineon and all other partners to maintain the momentum in jointly promoting GaN technology in the market alongside Chicony Power, making the power industry greener and cleaner.”
The post Infineon receives “GaN Strategic Partner of the Year” award from Chicony Power Technology appeared first on ELE Times.
RenewX 2024 – Fuelling India’s Renewable Energy momentum in the southern hub of Hyderabad
- 8th edition of the premier show by Informa Markets in India to be held on 26th and 27th April 2024 at HITEX Exhibition Centre
- RenewX 2024 will bring together over 5500 visitors, more than 150 exhibitors showcasing over 180 brands
Informa Markets in India, India’s leading B2B exhibitions organizer is gearing up to host the 8th edition of South India’s largest expo for renewable energy and electric vehicle market, RenewX. Scheduled to take place at Hall 1 & 3, HITEX Exhibition Centre, Hyderabad on 26th and 27th April 2024, the expo aims to bring together renewable energy and sustainable mobility professionals under one roof. It aims to set a growth trajectory for the sector, enabling networking, collaboration, and learnings from industry experts and thought leaders.
The show will attract a diverse audience including architects, real estate developers, commercial & industrial consumers, facility managers, energy consultants, independent power producers, EPCs, distributors/dealers, system installers and integrators, regulatory bodies, municipalities, local authorities and more.
Opening Doors to South India’s Renewable Energy HorizonRenewX 2024 is a gateway to discover various opportunities and build successful business relationships. Building on the success of last year’s event, the show is expected to welcome over 5500 visitors, and the presence of more than 150 domestic and international exhibitors including a few Chinese companies participating through their India operation team. Key exhibitors include top of the line organisations such as Premier Energies Ltd, Waaree Energies, Goldi Solar, Vikram Solar, Gautam Solar, Swelect Energy, Emmvee Solar, Jinko Solar, Rayzon Solar ,SMA Solar, Grew Energy, Credence Solar, Pahal Solar, , EVVO Solar, ICON Solar, MPL Group, Luminous Power Technologies, Microtek , Livguard Solar, Deye Inverter, Valeo products, Exide Industries, Polycab, and Impulse Green Energy, among many others.
A wide array of over 180 brands will be showcased with products and services spanning photovoltaic modules, hybrid systems, inverters, charge controllers, batteries, solar water heaters/cooling systems, solar pumps, testing and monitoring systems, project consultants, Wind-solar Hybrid Power System Integrators, EV & charging infra, assemblers and EPC players.
Emphasizing the significance of RenewX 2024, Mr. Yogesh Mudras, Managing Director, Informa Markets in India, said “India’s steadfast commitment to reducing carbon intensity by less than 45% by the decade’s end and achieving net-zero carbon emissions by 2070 underscores the imperative of carbon-free growth on our journey towards a $5 trillion economy. With solar PV capacity representing around 55% of our total renewable capacity, and the government’s unwavering focus on sustainable growth, solar energy emerges as a vital solution to meet our energy needs and ensure security, reaffirming India’s global leadership in renewable energy and dedication to sustainability. The recent approval of the Surya Ghar Yojana scheme for installing rooftop solar in 1 crore households further exemplifies India’s advancements in solar technology and manufacturing capabilities, cementing solar energy as a cornerstone of India’s sustainable future. Complemented by wind, biomass, EV, and hydropower, the nation’s focus on renewable energy has never been stronger.
RenewX 2024, set in the vibrant Southern hub of Hyderabad, will serve as a timely catalyst for elevating businesses and knowledge to new heights. As in previous editions, the RenewX conference, along with the exhibition, will foster a platform for understanding manufacturing challenges and collaboratively crafting solutions, with a special emphasis on South India’s unique context,” he further added.
A Comprehensive and Engaging eventTelangana State Renewable Energy Development Corporation will be the nodal agency at the show, facilitating a two-day conference featuring a CEO conclave and product showcase. The conference will comprise 7 sessions with over 40 speakers, discussing a wide array of topics such as Indian solar manufacturing, RE targets, solar technology advancements, net-zero and E-mobility, AgriPV, Bioenergy, and more. Distinguished dignitaries slated to speak include Sri Ajay Mishra, IAS, Director General, Renewable Energy Society of India (RESI) & (former) Spl. Chief Secretary, Govt of Telangana , Sri N. Janaiah, Vice Chairman & Managing Director, TSREDCO, Mr. Abhijeet Sinha, National Program Director Ease of Doing Business | Project Director- NHEV, DIISHA, Drone Pilot | President- CPOs of India, Mr Praveen Arora, Partner & Practice Lead- Energy & Infrastructure at BTG Advaya Mr. Amit Shukla, Senior VP and business Head, Energy Solutions, Luminous Power Technologies.;; Mr. Swapnil Wakade, Business Head – Rooftop Solar, Ecofy; Mr.Sumit Kumar – AVP Technology, Vikram Solar; Mr. Gaurav Kedia, Chairman, Indian Biogas Association; Mr. Rohit Dhar , COO, Emmvee Group, among others, will be sharing their valuable insights. The format would be a diverse spread of interactive channels like Confenrence Sessions, Multiple Workshops and Seminars and a Bioenergy Conference.
Industry Association & Knowledge Partners SupportThis year the expo garnered extensive support from partners and associations include TSREDCO (Telangana State Renewable Energy Development Corporation Ltd), IBA (Indian Bio-gas Association) , IGEF (Indo German Energy Forum), TELMA, TSEA (Telangana Solar Energy Association). The show’s knowledge partners include Frost & Sullivan, Wood Mackenzie, Yole Group, National Highway for EV and Ease of doing Business.
Driving India Towards a Sustainable Energy FutureIndia’s Renewable Energy Sector has experienced remarkable growth, driven by a convergence of factors including favourable Government Policies, cost reductions, increased investment, technological advancements, and heightened environmental awareness. Ranked as the 4th largest installed capacity for renewable energy globally, India’s solar energy sector has emerged as a major player in grid-connected power generation. With ambitious targets set to reduce the nation’s carbon intensity by less than 45% by the end of the decade, achieve 50% cumulative electric power installed by 2030 from renewables, and attain net-zero carbon emissions by 2070, India stands at the forefront of sustainable energy development. Steadfast in its commitment to prioritize solar power, India aims to surpass the 500 GW target before 2030, solidifying its global leadership in renewable energy and unwavering dedication to sustainability.
The post RenewX 2024 – Fuelling India’s Renewable Energy momentum in the southern hub of Hyderabad appeared first on ELE Times.
Research cooperation: LANXESS and IBU-tec to develop iron oxides for LFP battery material
- Collaboration aims to improve performance of LFP cathode material
- Reduced carbon footprint of batteries through use of local raw materials
- Focus on European value chain for LFP batteries
Specialty chemicals company LANXESS and battery materials manufacturer IBU-tec advanced materials have entered into a research cooperation in the battery sector. The goal of the two German companies is to develop innovative iron oxides for the production of cathode material for LFP batteries and thus increase the performance of this battery type. The companies aim to optimize the electrochemical properties of LFP batteries, such as energy density, charging speed and number of charging cycles.
More and more car manufacturers are increasingly relying on LFP (lithium/iron/phosphate) batteries for their e-vehicles – especially for volume models. Compared to NMC (nickel/manganese/cobalt oxide) and NCA (nickel/cobalt/aluminum oxide) cell chemistry systems, LFP technology offers cost advantages of up to 50 percent and promises safer use, as the system makes the batteries virtually impossible to ignite.
Strengthening European value chainsThe demand for LFP in Europe is expected to grow by 20 percent per year until 2030. So far, however, this demand has been met almost exclusively by non-European suppliers. With their development, both companies aim to contribute to the establishment of an independent, robust value chain in the European LFP battery market, while at the same time reducing the carbon footprint of batteries.
IBU-tec, based in Weimar, Germany, is currently the only European manufacturer of LFP cathode material. LANXESS operates the world’s largest plant for the key raw material iron oxide in Krefeld-Uerdingen. The company has almost 100 years of experience in developing this material and can supply iron oxide particles for LFP batteries in the required size, purity, morphology and quantities.
Michael Ertl, Head of the Inorganic Pigments business unit at LANXESS, said: “As IBU-tec is currently the only European manufacturer of LFP cathode materials, the company is the ideal partner for us to develop the new material, which is a key component for batteries in e-cars and stationary energy storage systems. This is an important contribution to sustainability and the development of a European value chain in the field of battery materials.”
Jörg Leinenbach, CEO of IBU-tec, said: “With LANXESS, we are gaining one of the largest, globally positioned chemical companies as a strong partner in the battery sector. With the joint product development we will combine our expertise and together we will drive the development of the European LFP battery market and establish an independent value chain. We see this cooperation as an important step towards opening up the market. IBU-tec will inform about further material developments in the battery sector with new application possibilities in January.”
LANXESS: Wide range of solutions for electromobilityIn addition to key ingredients for LFP precursors, LANXESS offers many other solutions for electromobility and the battery industry, including raw materials for electrolytes, battery coolants, flame retardants for plastic components in electric vehicles and charging infrastructure, and orange dyes for coloring high-voltage components.
The post Research cooperation: LANXESS and IBU-tec to develop iron oxides for LFP battery material appeared first on ELE Times.
Light + Building 2024: Fulminant innovation show provides a stage for sustainability and efficiency in buildings
The modern building is intelligent, connected and as a result saves energy. In combination with alternative energy sources and efficient lighting solutions, emissions in the building sector can be drastically reduced. This makes a significant contribution to achieving climate targets. 2,169 exhibitors presented the latest developments in building technology and trends in innovative lighting design at Light + Building in Frankfurt am Main from 3 to 8 March 2024. Over 151,000 visitors travelled to the world’s leading trade fair for lighting and building-services technology.
“The atmosphere at the exhibitors’ booths, in the halls and throughout the exhibition grounds was simply fantastic. We are extremely pleased that so many exhibitors and visitors, as well as our long-standing partners, have continued the success story of the world’s leading trade fair for lighting and building-services technology in 2024,” summarises Wolfgang Marzin, President and Chief Executive Officer of Messe Frankfurt. He adds: “With the switch to renewable energy sources, greater efficiency and sustainability in buildings, the industry has key goals on its agenda. That’s why they used the platform intensively, especially in the first few days, to present and discover innovations and drive forward key topics. After all, if we want to achieve the climate protection goals, the building sector is an essential milestone. It is unfortunate that the rail and air transport strikes have already affected Messe Frankfurt’s third leading international event since the beginning of the year.”
Buildings of tomorrow and inspiring lighting solutionsKey topics are the electrification and digitalisation of homes and buildings in order to reduce emissions and reuse raw materials. At Light + Building, the industry presented the digital and electrotechnical infrastructure for this and, on this basis, showcased solutions for dynamic power control, energy storage systems and applications for connected security. One growing area is the range of e-mobility and charging infrastructure as well as innovations and products for decentralised energy supply systems and components.
Light plays an important role in the architecture of tomorrow. At Light + Building 2024, 65 per cent of exhibitors belonged to this sector. They presented high-quality lighting solutions for indoor and outdoor areas as well as dynamic room concepts. Modern LED installations ensure contemporary efficiency and either blend harmoniously into the architecture or emphasise the design elements. Lighting is to provide maximum visual comfort in all living and working environments. Thanks to the materials used, Acoustic Lighting combines a pleasant lighting atmosphere with sound-absorbing functions. Sustainability plays an essential role in both the materials used and the manufacturing processes. Many manufacturers design luminaires in a way that the raw materials used can be recycled at the end of their useful life.
Light + Building 2024 in figuresThe high-quality, extensive and international portfolio of lighting and building-services technology impressed the visitors. 95 per cent of them were extremely satisfied with what was on display and stated that they had achieved 93 per cent of their trade fair attendance targets. The most came to the innovation meeting point from Germany, China, Italy, the Netherlands, France, Switzerland, Belgium, Austria, the UK, Spain and Poland. They came from a total of 146 countries – including, for example, India, the USA, the United Arab Emirates, Australia, Brazil and Singapore. The degree of internationality was thus 51 per cent. The level of internationality among the 2,169 exhibitors was also high at 76 per cent.
Meeting place for the social media communityThe social media community also found its home at Light + Building. On 3 and 4 March, the leading content creators in the lighting and building-services technology sector gathered for the Power Creator Days. In addition to live podcasts, expert talks and case studies, visitors had the chance to pedal for a good cause and work together towards a high energy target. A total of 1,510 minutes were cycled on the six fitness bikes. The sponsors will convert the result into a cash donation for the Leberecht Foundation, which Messe Frankfurt will double. The exact amount will be announced on social media further to Light + Building.
The next Light + Building will take place from 8 to 13 March 2026 in Frankfurt am Main.
Voices of the industry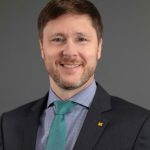
Alexander Neuhäuser, General Manager ZVEH, Central Association of the German Electrical and Information Technology Trades, says that “Light + Building demonstrates how sector coupling can succeed through the necessary connectivity. The electrical trades integrate photovoltaics, storage, electromobility and heat pumps. They show how the energy industry requirements for controllable consumption devices (SteuVE) can be met and thus take account of the current transformation process. The good atmosphere at this year’s Light + Building 2024 was also noticeable at the joint stand of the electrical trades, which was very busy on all days of the event. The traditional partners’ evening was also a complete success, bringing together the partners of the electrical trades and the industry leaders. We were particularly pleased that so many young people once again took the opportunity to visit the E-House and the workshop street and gain an impression of what is feasible with smart and intelligently connected building automation.”
Wolfgang Weber, CEO, ZVEI, Electro and Digital Industry Association, is of the opinion that, “In the context of climate goals and the economic situation of urgently creating more affordable living space in Germany, technologies are increasingly coming into focus. The exhibiting companies at Light + Building have impressively demonstrated how easily well-designed climate protection can even lead to greater economic efficiency in the operation of houses, buildings and entire neighbourhoods.
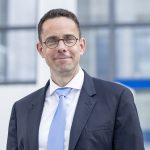
This requires the right solutions, especially from the electrical and digital industry, such as heat pumps, controllable lighting, charging points and an energy management system. This is relevant – not just in Germany and Europe, but worldwide. Light + Building is the right place to present innovative, climate-friendly technologies and solutions and to engage in dialogue with trade visitors from Germany and abroad.”
The post Light + Building 2024: Fulminant innovation show provides a stage for sustainability and efficiency in buildings appeared first on ELE Times.
Microchip Acquires ADAS and Digital Cockpit Connectivity Pioneer VSI Co. Ltd. to Extend Automotive Networking Market Leadership
Acquisition adds ASA Motion Link technology to Microchip’s broad Ethernet and PCIe automotive networking portfolio to enable next-generation software-defined vehicles
Microchip Technology Inc. has announced the completed acquisition of Seoul, Korea-based VSI Co. Ltd., an industry pioneer in providing high-speed, asymmetric, camera, sensor and display connectivity technologies and products based on the Automotive SerDes Alliance (ASA) open standard for in-vehicle networking (IVN). The terms of the transaction are not disclosed.
The market size of automotive radar, camera and LiDAR modules is expected to grow by greater than two times between 2022 to 2028 to $27B in revenue, according to Yole Group[1]. This anticipated growth is driven by the increased adoption of Advanced Driver Assistance Systems (ADAS), in-cabin monitoring, safety and convenience features (e.g., 360-degree surround view, e-mirrors) and multi-screen digital cockpits for next-generation software-defined vehicles (SDV). These applications will require more highly asymmetric raw data and video links and higher bandwidths, making current, proprietary serializer/deserializer (SerDes) based solutions no longer adequate, both commercially and technically. In response to these developments, the Automotive SerDes Alliance (ASA) was formed in 2019 and released the first open-standard ASA Motion Link (ASA-ML) specifications.
“This acquisition brings VSI’s knowledgeable team, their market traction and ASA Motion Link technologies and products to Microchip’s expansive automotive networking portfolio to better serve the ADAS megatrend we are focused on,” said Mitch Obolsky, senior vice president of Microchip’s automotive products, networking, and data centre business units. “As the industry converges around three primary IVN pillars – Ethernet, PCIe and ASA Motion Link, camera and display connectivity is one of the fastest growing and largest IVN markets. With VSI, Microchip can now offer products that span all three pillars and also provide automotive security, microcontrollers, motor control, touch and power management solutions to our customers to enable their next-generation software-defined vehicle architectures.”
Today, ASA has over 145 members, including Microchip who is a promoter member. With 11 automotive manufacturers including BMW, GM, Ford, Stellantis and Hyundai-Kia Motors Corporation, the Alliance also includes an ecosystem ranging from Tier 1 suppliers, semiconductor and imager vendors, to test and compliance houses. In addition to being an open standard, ASA-ML brings link layer security and scalability to support 2 Gbps to 16 Gbps line rates. Furthermore, the upcoming specification update will enable ASA-ML to support Ethernet-based architectures.
“Microchip Technology is an established and trusted market leader in automotive networking known for their automotive quality and robust supply chain, and our team is excited to join them to address the growing ADAS and digital cockpit connectivity market,” said Steve Kang, CEO of VSI Co. Ltd. “VSI is a leader in the development of ASA-ML products and was the first to introduce products to the market. Our standards-compliant chipsets are being evaluated by car manufacturers worldwide. We recently collaborated with BMW in a proof of concept to showcase ASA-ML and our product readiness. This acquisition brings together two organizations with a shared commitment to advancing technology through innovation. We look forward to successfully deploying our solutions in production vehicles for years to come.”
In March 2024, BMW Group announced at the Automotive Ethernet Congress in Munich they would shift to using standardized ASA-ML for the upcoming start of productions. BMW has always been at the forefront of in-vehicle networking innovation and strongly believes in leveraging standardized technologies in their vehicle architectures and now also their video architecture.
[1] Sources: LiDAR for Automotive – Radar for Automotive – Status of the Camera Industry – Yole Intelligence, 2023.
The post Microchip Acquires ADAS and Digital Cockpit Connectivity Pioneer VSI Co. Ltd. to Extend Automotive Networking Market Leadership appeared first on ELE Times.
Vishay Intertechnology Industrial-Grade TRANSZORB and Automotive Grade PAR TVS Deliver Peak Pulse Power of 600 W in DFN3820A Package
Featuring Compact 3.8 mm by 2.0 mm Footprint, Low 0.88 mm Profile, and Wettable Flanks, Space-Saving Devices Offer Operating Temperatures to +185 °C
Vishay Intertechnology, Inc. today introduced four new series of surface-mount industrial-grade TRANSZORB and automotive-grade PAR transient voltage suppressors (TVS) in the low profile DFN3820A package with wettable flanks. Providing space-saving solutions for automotive, computer, consumer, and industrial applications, the 6DFNxxA, 6DFNxxxCA, T6NxxA, and T6NxxxCA offer peak pulse power of 600 W at 10/1000 μs and low leakage current down to 1 μA.
The first package in Vishay’s new Power DFN family, the DFN3820A features a compact 3.8 mm by 2.0 mm footprint and an extremely low typical height of 0.88 mm, allowing the Vishay General Semiconductor TVS to make more efficient use of PCB space. Footprint-compatible with the SMP (DO-220AA) package, the DFN3820A is 85 % smaller than the SMB (DO-214AA) and 42 % smaller than the SlimSMAW (DO-221AD), but it keeps peak pulse power dissipation with a 10/1000 μs waveform at 600 W.
The devices released are designed to protect sensitive electronic equipment against voltage transients induced by inductive load switching and lightning. The 6DFNxxA and 6DFNxxxCA TRANSZORB TVS will be used for signal line protection in server power modules, digital media controllers, and AV signal extenders for computer and consumer applications, in addition to industrial robot control boards, process/flow control instruments, and automation systems.
AEC-Q101 qualified and offering high-temperature operation to +185 °C, the T6NxxA and T6NxxxCA PAR TVS are intended for automotive load dump protection. Typical applications will include advanced driver assistance (ADAS), battery management (BMS), electric power steering (EPS), and infotainment systems; central control units; on-board chargers (OBC); DC/DC converters and traction inverters; and electrical motor drives.
The TVS offer excellent clamping capability with a maximum clamping voltage from 16.7 V to 70.1 V for the 6DFNxxA and T6NxxA, and 16.7 V to 137 V for the 6DFNxxxCA and T6NxxxCA. The wettable flanks of their DFN3820A package allow for automatic optical inspection (AOI), eliminating the need for an X-ray inspection. Ideal for automated placement, the rectifiers offer an MSL moisture sensitivity level of 1, per J-STD-020, LF maximum peak of 260 °C. The devices are RoHS-compliant and halogen-free, and their matte tin-plated leads meet the JESD 201 class 2 whisker test.
Device Specification Table:
Series |
6DFNxxA |
6DFNxxxCA |
T6NxxA |
T6NxxxCA |
Automotive Grade |
No |
No |
Yes |
Yes |
VBR (V) |
12 to 51 |
12 to 100 |
12 to 51 |
12 to 100 |
VWM (V) |
10.2 to 43.6 |
10.2 to 85.5 |
10.2 to 43.6 |
10.2 to 85.5 |
Max. reverse leakage at VWM (mA) |
1.0 to 2.0 |
|||
PPPM (10/1000 μs) (W) |
600 |
|||
Max. clamping voltage (V) |
16.7 to 70.1 |
16.7 to 137 |
16.7 to 70.1 |
16.7 to 137 |
TJ max. (°C) |
175 |
175 |
185 |
185 |
Polarity |
Unidirectional |
Bidirectional |
Unidirectional |
Bidirectional |
Package |
DFN3820A |
|||
Circuit configuration |
Single |
The post Vishay Intertechnology Industrial-Grade TRANSZORB and Automotive Grade PAR TVS Deliver Peak Pulse Power of 600 W in DFN3820A Package appeared first on ELE Times.
Ceremorphic Driving Vision to Develop Sustainable Product Portfolio Across Sectors
Ceremorphic is doing great business and quality development in the areas of supercomputing, datacentre, life sciences, automotive, robotics, and metaverse processing. As the world moves towards a highly advanced computing era, Ceremorphic is leading many channels in developing high-tech computing and engineering systems. Their technical excellence covers a vast portfolio including multi-thread processors, security processing, machine learning processors, analog computing, reliable circuits, 3D interconnects, graph neural processing, programmable logic, and low-power memory.
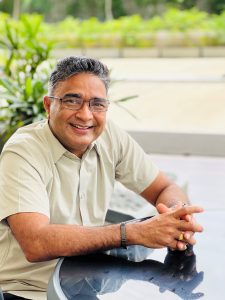
Rashi Bajpai, Sub-Editor at ELE Times interacted with Dr. Venkat Mattela, Founder & CEO, of Ceremorphic about the company’s vision and technical competence, and also touched upon topics of Make in India and ESG norms.
This is an excerpt from the conversation.
ELE Times: Please throw some light on Ceremorphic’s recent development of the ultra-low power supercomputer chip built in TSMC 5nm.
Venkat Mattela: Designing products with optimal energy consumption is a challenge we foresee for the semiconductor industry for the next few decades especially with the advent of carbon neutrality mandates. As supercomputing innovations burgeon and data centers consume ever more energy, within a decade they will consume a significant part of the total energy generation in the world. This was the catalyst behind our mission to design chips with ultra-low energy consumption. Our breakthrough innovation is not just in advanced nodes like 5nm and 3nm, but we are also broadening our reach to other paradigms to minimize task workloads alongside semiconductor technology optimizations. Having taped out our 5nm chip in October 2022 and validated key technologies for our upcoming 3nm device, we’re proud of how far we’ve come in addressing these challenges. Equipped with advanced features like multi-thread processing and custom connectivity interfaces, our chips cater to modern applications’ rigorous demands, from AI model training to drug discovery. We’re excited to continue pushing the boundaries of technology and remain committed to creating solutions that make a meaningful impact.
ELE Times: In the latest development under the “Make in India” policy, India is set to begin manufacturing equipment for semiconductor manufacturing. In this regard, what in your view, are India’s major contributions to the semiconductor industry besides cutting-edge R&D? Also help us understand the challenges with physical manufacturing.
Venkat Mattela: In addition to cutting-edge R&D, India’s contributions to the semiconductor industry extend across various domains, showcasing the nation’s growing significance in this field. Firstly, India serves as a hub for semiconductor design and engineering services, with numerous companies specializing in chip design, verification, and testing. These capabilities play a crucial role in the global semiconductor supply chain, contributing to the development of innovative semiconductor products.
We also boast a robust ecosystem for semiconductor software development, encompassing tools and platforms for chip design, simulation, and optimization. This is complemented by our vast pool of skilled engineers and technologists that form a rich talent pool sought after by semiconductor companies worldwide. Moreover, initiatives like the Skill India program aim to enhance the employability of Indian youth in high-growth sectors such as semiconductors, fostering a skilled workforce for the industry’s future needs.
However, despite these contributions, India faces several challenges in physical manufacturing. One key challenge is the need for robust infrastructure and logistical support. Semiconductor fabrication facilities require state-of-the-art equipment, cleanroom environments, and reliable power and water supply, which may be lacking in certain regions.
Additionally, establishing a consistent supply chain for raw materials, chemicals, and equipment components is crucial to reduce reliance on imported materials and prevent disruptions. To scale up manufacturing, it is crucial to present ourselves as an ideal candidate for manufacturing thereby attracting investments in research, infrastructure, and workforce development.
While initiatives like the “Make in India” policy aim to do this, sustained efforts are needed to overcome regulatory barriers, attract foreign investment, and push for innovation-driven growth in the sector.
ELE Times: What potential collaborations can be explored between the renewable energy sector and semiconductor fabrication plants to establish ESG norms and sustainable practices in manufacturing?
Venkat Mattela: Renewable energy should not be viewed as merely an option, but rather as a standard practice across all industries. Given the semiconductor industry’s substantial energy consumption, it is crucial for us to take the lead in adopting renewable energy sources. Collaborations between the renewable energy sector and semiconductor fabrication plants play a pivotal role in establishing robust ESG norms and sustainable manufacturing practices.
By integrating renewable energy sources such as solar, wind, and hydroelectric power into semiconductor fabrication plants we can significantly reduce carbon emissions and environmental impact. Research and development efforts aimed at enhancing energy efficiency in semiconductor fabrication, such as advanced cooling systems, energy-efficient equipment, and optimized manufacturing processes, can yield significant environmental benefits while also improving operational efficiency and cost-effectiveness. Additionally, encouraging partnerships between renewable energy providers and semiconductor companies can facilitate the development of innovative solutions for energy storage and management. Technologies like battery storage systems and smart grid integration enable semiconductor facilities to store excess renewable energy and optimize its usage based on demand, thereby maximizing energy efficiency and grid stability. By leveraging renewable energy sources, emphasizing innovation, and promoting sustainability across the supply chain, we can contribute to a more environmentally conscious and socially responsible semiconductor industry.
ELE Times: What would be your insights and recommendations for the policymakers navigating the challenges of establishing India as a semiconductor hub?
Venkat Mattela: We are making significant strides in positioning India as a global R&D hub, thanks to our supportive policies and initiatives that prioritize top-tier institutions and foster innovation, particularly in AI. However, transitioning into a semiconductor manufacturing hub requires additional effort and time. A crucial starting point would be to build a semiconductor manufacturing facility, which is doable and requires very dedicated engineering leadership to action. Fortunately, the current government’s policies are highly encouraging and provide India an ideal path to make substantial progress in this direction within the coming decade.
ELE Times: Looking at Ceremorphic’s technology portfolio, help us understand its role in addressing the crucial needs of the high-performance computing era.
Venkat Mattela: Generative AI has played a critical role in enabling various technological applications to efficiently adopt Artificial Intelligence (AI) and supercomputing is the catalyst in training these AI models. At Ceremorphic, we embarked on this journey six years ago with a primary objective of developing ultra-low-energy, low-power supercomputing solutions. While few companies prioritized low-energy supercomputing at the time, it has now become an industry standard. With a portfolio boasting over 200 patented core technologies in this field, we have certainly has played a part in driving this shift. Our efforts in advancing low-energy supercomputing have had far-reaching implications, especially in the life sciences and pharmaceutical space.
We recently introduced our new platform, BioCompDiscoverX, which promises to revolutionize drug development by significantly accelerating the process, reducing costs, and enhancing efficacy. Furthermore, reiterating our commitment to high-performance computing in an energy-efficient manner, our upcoming chip will be manufactured using TSMC’s cutting-edge 3nm node.
ELE Times: What extra efforts and measures are necessary on the grassroots level to further elevate and enhance skill development across various sectors and emerging technologies?
Venkat Mattela: Progress at the grassroot level hinges on the coming together of supportive policies and robust funding infrastructures. As we enter a pivotal decade of innovation, we are witnessing promising advancements in these areas within India. Positioned in our golden era, we have the privilege of seizing numerous opportunities to leave a significant impact on the upcoming technological era.
Advanced universities are already leading the way by implementing specialized curriculums tailored to new technologies. But, to ensure widespread skill development, we must distil key learnings and core concepts and integrate them into the lower education levels to establish a solid foundation for future generations. This approach must go beyond theoretical knowledge and touch upon practical demonstrations of concepts. By encouraging innovation ideation from an early stage, we can sustainably cultivate a culture of creativity that will propel us to the forefront of every emerging technology.
The post Ceremorphic Driving Vision to Develop Sustainable Product Portfolio Across Sectors appeared first on ELE Times.
Anritsu Company Expands Inline Sensor Family
Anritsu Company is proud to announce the launch of our new inline power sensor MA24103A which is designed to measure accurate Peak and True-RMS average power measurements from 25 MHz to 1 GHz and 2 mW to 150 W power range.
Several applications demand accurate peak and average power measurements well below the frequency range of 1 GHz. Agencies in Public Safety, Avionics (air traffic control and repair stations), and Railroads, etc. must maintain critical communications between the control centres and the vehicles. The slightest error in making measurements or maintaining a communication network in these markets could risk public safety or even have fatal consequences.
The advantage of lower frequencies is that they can propagate a longer distance and maintain communication with fast-moving vehicles. Normally, at lower frequencies, the power of the transmitting signal is in the range of watts, which makes the MA24103A more suited for these types of applications.
This highly accurate, Inline Peak Power Sensor communicates with a PC via USB or with an Anritsu handheld instrument equipped with the high-accuracy power meter option 19.
Some of the main markets that benefit from this low-frequency Inline Power Sensor include:
- Broadcast Network and Manufacturer: Lab performance accuracy and low insertion loss over a wide temperature range (0 ºC to 55 ºC), making it perfect for field applications.
- Railroads: to evaluate various systems like Positive Train Control Systems, End of Train (EOT) signals, automated train control systems, and FM voice base stations.
- Avionics: such as Civil and Military Airports for beacon testing, surveillance radar testing, localizer, and marker testing.
The post Anritsu Company Expands Inline Sensor Family appeared first on ELE Times.
Renesas Expands Quick Connect Studio with Real-Time Code Customization, Remote Debugging and Broad Portfolio of Supported Devices
Industry’s First-Ever Cloud-based System Development Tool Enables Rapid Prototypes and Co-Optimization of Software and Hardware
The post Renesas Expands Quick Connect Studio with Real-Time Code Customization, Remote Debugging and Broad Portfolio of Supported Devices appeared first on ELE Times.
Exploring Process Scenarios to Improve DRAM Device Performance
In the world of advanced semiconductor fabrication, creating precise device profiles (edge shapes) is an important step in achieving targeted on-chip electrical performance. For example, saddle fin profiles in a DRAM memory device must be precisely fabricated during process development in order to avoid memory performance issues. Saddle Fins were introduced in DRAM devices to increase channel length, prevent short channel effects, and increase data retention times.
Critical process equipment settings like etch selectivity or the gas ratio of the etch process, can significantly impact the shape of fabricated saddle fin profiles. These process and profile changes have significant impact on DRAM device performance. It can be challenging to explore all possible saddle fin profile combinations using traditional silicon testing, since wafer-based testing is time-consuming and expensive. To address this issue, virtual fabrication software (SEMulator3D) can be used to test different saddle fin profile shapes without the time and cost of wafer-based development.
In this article we review an example of using virtual fabrication for DRAM saddle fin profile development. We also assess DRAM device performance under different saddle fin profile conditions. This methodology can be used to guide process and integration teams in the development of process recipes and specifications for DRAM devices.
The Challenge of Exploring Different ProfilesImagine you are a DRAM process engineer, and have received nominal process conditions, device specifications, and a target saddle fin profile for a new DRAM design. You want to explore some different process options and saddle fin profiles to improve the performance of your DRAM device. What should you do? This is a common situation for integration and process engineers during the early R&D stages of DRAM process development.
Traditional methods of exploring saddle fin profiles are difficult and sometimes impractical. These methods involve the creation of a series of unique saddle fin profiles on silicon wafers. The process is time-consuming, expensive, and in many cases impractical, due to the large number of scenarios that must be tested.
One solution to these challenges is to use virtual fabrication. SEMulator3D allows us to create and analyze saddle fin profiles within a virtual environment and to subsequently extract and compare device characteristics of these different profiles. The strength of this approach is its ability to accurately simulate the real-world performance of these devices, but to do so faster and less-expensively than using wafer-based testing.
Let’s dive into the methodology behind our approach.
Creating Saddle Fin Profiles in a Virtual EnvironmentFirst, we input the design data and process flow (or process steps) for our device in SEMulator3D. The software can then generate a “virtual” 3D DRAM structure and provide a visualization of saddle fin profiles (Figure 1). In Figure 1(a), a full 3D DRAM structure including the entire simulation domain appears. To enable detailed device study, we cropped a small portion of the simulation domain from this large 3D area. In Figure 1(b), we extracted a cross sectional view of the saddle fin structure, which can be modified by varying a set of multi-etch steps in the process model. The section of the saddle fin we want to modify is identified as the “AA” (active area). We can finely tune the etch taper angle, AA/fin CD, fin height, taper angle, and additional nominal device parameters to modify the AA profile.
Using the structures, we built in SEMulator3D, we next assign dopants and ports to the simulated structure and perform electrical performance evaluation. Accurately assigning dopant species and defining dopant concentrations within the structure is critical to ensuring the accuracy of our simulation. In Figure 2(a), we display a dopant concentration distribution generated in SEMulator3D.
Ports are contact points in the model, which are used to apply or extract electrical signals during a device study. Proper assignment of the ports is very important. Figure 2(b) provides an example of port assignment in our test DRAM structure. By accurately assigning the ports and dopants, we can extract the device’s electrical characteristics under different process scenarios.
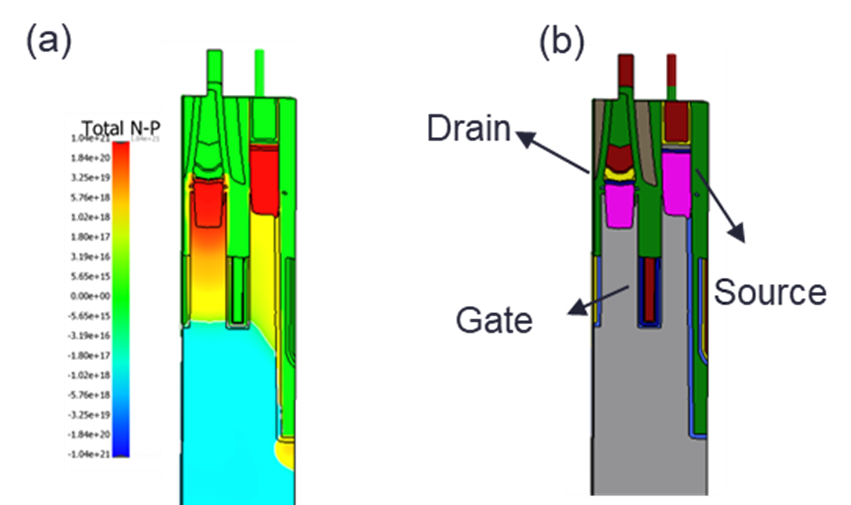
It is important to ensure that our simulation models match real world results. We can validate our model against cross-sectional images (SEM or TEM images) from an actual fabricated device. To ensure our simulated device matches the behavior of an actual manufactured chip, we can create real silicon test wafers containing DRAM structures with different saddle fin profiles.
To study different saddle fin profiles, we use different etch recipes on an etch machine to vary the DRAM wordline etch step. This allows us to create specific saddle fin profiles in silicon that can be compared to our simulated profiles. A process engineer can change etch recipes and easily create silicon-based etch profiles that match simulated cross section images, as shown in Figure 3.
In this case, the engineer created a nominal (Process of Record) profile, a “round” profile (with a rounded top), and a triangular shaped profile (with a triangular top). This wafer-based data is not only used to test electrical performance of the DRAM under different saddle fin profile conditions, but can also be fed back into the virtual model to calibrate the model and ensure that it is accurate during future use.
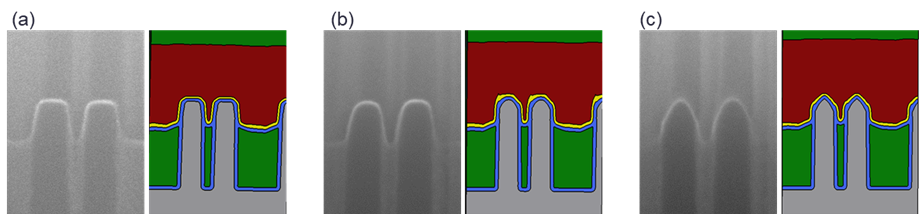
In the final stage of our study, we review the electrical simulation results for different saddle fin profile shapes. Figure 4 displays simulated electrical performance results for the round profile and triangular saddle fin profile. For each of the two profiles, the value of the transistor Subthreshold Swing (SS), On Current (Ion), and Threshold Voltage (Vt) are displayed, with the differences shown. Process integration engineers can use this type of simulation to compare device performance using different process approaches. The same electrical performance differences (trend) were seen on actual fabricated devices, validating the accuracy and reliability of our simulation approach.
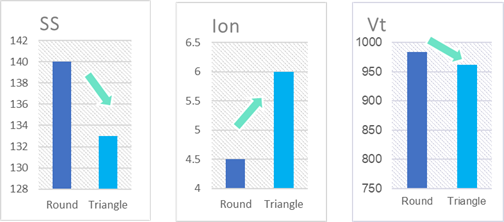
SEMulator3D provides numerous benefits for the semiconductor manufacturing industry. It allows process integration teams to understand device performance under different process scenarios and lets them easily explore new processes and architectural opportunities.
In this article, we reviewed an example of how virtual fabrication can be used to assess DRAM device performance under different saddle fin profile conditions. Figure 5 displays a summary of the virtual fabrication process and how we used it to understand, optimize, and validate different process scenarios.
Virtual fabrication can be used to guide process and integration teams in the development of process recipes and specifications for any new memory or logic device and to do so at greater speed and lower cost than silicon-based experimentation.
The post Exploring Process Scenarios to Improve DRAM Device Performance appeared first on ELE Times.
TATA in Partnership with Satellogic Launches First Made in India Military Satellite
Tata Advanced Systems Ltd, a subsidiary of TATA Sons, in collaboration with Satellogic has successfully launched India’s first private sector-built sub-metre resolution earth observation satellite, TSAT-1A from the Kennedy Space Centre, Florida through SpaceX’s Falcon 9 rocket on April 7.
The TSAT-1A satellite assembled at TASL’s Karnataka facility represents a significant milestone in space technology. Its deployment as part of SpaceX’s inaugural Bandwagon flight, which also deployed a total of 11 satellites, underscores its importance in the realm of space exploration and satellite deployment.
The satellite is anticipated to bring about a paradigm shift in satellite imaging due to its high-resolution capabilities and rapid data delivery. Its configuration in low-earth orbit, coupled with its lightweight design of less than 50 kg, positions it as a versatile asset capable of serving a wide range of applications. Its potential impact on various sectors including defense and communication, makes it a pivotal development in the field of space technology.
The post TATA in Partnership with Satellogic Launches First Made in India Military Satellite appeared first on ELE Times.
Semiconductors beyond nanometers
SCHUMACHER | Infineon Technologies AG
In today’s world, semiconductors are essential components of our everyday lives and the backbone of our economies. From the devices we use to communicate to the machines that power our factories, semiconductors are the building blocks that enable digitalization and decarbonization. However, public and policy debates about semiconductors often focus solely on their smallest feature size, measured in nanometers, which fails to capture the full complexity and importance of these high-tech goods.
At Infineon, we have developed a simple yet powerful way to understand semiconductors – our “Semiconductor Tree.” This intuitive structure provides a framework for discussing the different branches of the semiconductor industry, from power semiconductors to memory chips and microcontrollers. By exploring each branch of the tree, we can gain a better understanding of the unique properties and specialized industries that each one represents.
First transistor (1947)At the core of the semiconductor industry is the transistor switch, which was first demonstrated in 1947 by a team of researchers at AT&T Bell Labs. Since then, we have seen an exponential miniaturization roadmap, which has led to microprocessors that contain billions of transistors. However, this race to shrink transistors and integrate them as densely as possible into an Integrated Circuit (IC) requires extreme ultraviolet light and the most complex machines ever manufactured by mankind.
Power semiconductorsNotably, one branch of the semiconductor industry has focused on optimizing the individual transistor for faster switching and/or switching of ever-higher electrical power. These are known as power semiconductors and are becoming increasingly important as societies and economies move towards electrification as a means to decarbonize. Power semiconductors require sophisticated control of the underlying technologies and processes, and leading companies often control much of the value chain in-house.
Infineon’s power semiconductor business is an example of an Integrated Device Manufacturer (IDM), with major manufacturing sites in Germany, Austria, and Malaysia.
Memory, microcontrollers/microprocessors, and analog/mixed-signalAnother path to optimizing the transistor was to standardize it as much as possible, shrink it, and pack it as densely as possible. This gave rise to other branches in the semiconductor tree, including memory, microcontrollers, and microprocessors. Each of these branches has its unique properties, requirements, and industries. For instance, memory semiconductors allow storage and read-out of information in the form of electrical signals.
Microcontrollers, on the other hand, control everyday devices like washing machines, cars, airplanes, and industrial control systems. Analog/mixed-signal semiconductors act as the interface between our real world and the digital world, processing analog electrical signals like WiFi, Bluetooth, or radar chips for autonomously driving cars. Since analog signal processing doesn’t follow the same shrink path as analog, manufacturing analog/mixed signal today is often done at a sweet spot of 130-90nm.
Final ThoughtsThe world of semiconductors is vast and diversified, and it is crucial to have a more nuanced understanding of the importance and development of the respective industries. Semiconductors are essential building blocks for our personal lives, modern societies, and economies, and their importance will only grow as we continue to digitize and decarbonize.
As such, it is no surprise that semiconductors are at the center of global politics. By understanding the complexity and importance of semiconductors, we can have more precise discussions about their role in shaping our world.
The post Semiconductors beyond nanometers appeared first on ELE Times.
Examining an Air Filter with a RANS Turbulence Model
Joseph Carew | Comsol
HVAC systems do more than provide the smooth, chilled air that flows when the temperature outside rises. Within these systems, air moves through filters to ensure high air quality. With clean air at stake, modeling and simulation can be used to gain an in-depth understanding of the physics behind the behavior of air as it moves through a filter…
Modeling an Air FilterThe filters within HVAC systems rely on a material (often fiberglass or cotton folds) capable of straining the air and catching particulates like dust, pollen, and bacteria. These materials impact the flow of the air, catching the unwanted particulates while simultaneously allowing the filtered air to flow through. Modeling these devices and the turbulent flow they induce allows for determining the effectiveness of different materials when they are used for filters, helping designers to narrow down the material options before investing in real-life, experimental versions.
In this blog post, we will look at a common air filter geometry (shown below) as our example.
Modeling this air filter begins with the CFD Module, an add-on product to the COMSOL Multiphysics® software, which enables users to create Reynolds-averaged Navier–Stokes (RANS) turbulence models in open and porous domains. In this example, the air filter is modeled as a highly porous domain with 90% of the material occupied by cylindrical pores with a diameter of .1 mm. The support of the air filter is represented by a frame with no-slip walls. For this example, we employed the Turbulent Flow, k-ω interface because of its accuracy for models with many walls, including no-slip walls. (An in-depth look at the model setup can be found in the model documentation, which can be accessed via the button at the end of this blog post.)
Evaluating the ResultsSolving the model allows for visualizing the change in turbulence, velocity, and pressure as air moves toward, through, and past the filter. The computation begins with the air moving toward the filter (purple in the image below). When the air passes through the filter, the interstitial velocity increases (although the porous-averaged velocity remains constant), resulting in an increase in turbulence kinetic energy. Additionally, there is an abrupt pressure drop due to the increase in velocity and the increased friction and pressure losses, which stem from the high number of wall surfaces. As for the behavior of the air as it moves away from the filter, the frame of the filter prevents the air from moving freely, instead causing downstream wakes of air.
The visualization of the air moving through the filter can be used to conclude whether or not the filter will remove contaminants from the air. To confirm this conclusion, the solution can be evaluated with different slice plots. One of the slice plots for this example indicates that the velocity of the air is most impacted by the porous air filter and the frame and that it homogenizes through the wake region. A slice plot measuring the turbulence kinetic energy shows that the turbulence kinetic energy peaks noticeably within the filter and attains typical values on the no-slip walls.
In general, the model points to a pressure drop and a dramatic increase in turbulence within the filter, creating perturbations in velocity perpendicular to the main direction of the flow thus also increasing the probability of the particles to collide with the pore walls and stay there. In other words, the increase in turbulence provides the mixing required for filtering out the unwanted particulates, which otherwise would flow through the pores undisturbed.
The post Examining an Air Filter with a RANS Turbulence Model appeared first on ELE Times.
Broadcom brings together two proven portfolios to deliver complete hybrid cloud cybersecurity
Rob Greer, Vice President and General Manager, Enterprise Security Group, Broadcom
Merging Carbon Black with Symantec forms the new Enterprise Security Group at BroadcomIt is clear, there is no let-up in cyberattacks, so the timing could not be better for Broadcom to bring Carbon Black and Symantec together. These engineering-first, innovation-centric brands are both committed to delivering proven cybersecurity solutions and support built to meet the unique and highly complex challenges of the largest and most heavily regulated enterprise customers and partners. Broadcom will make significant investments in both brands, and continue to offer both portfolios under the Enterprise Security Group business unit. Our joint mission is to help secure the world’s largest and most advanced enterprises.
Symantec’s portfolio, with some of the best security technology and research in the world, concentrates on data and network protection, while Carbon Black’s complimentary portfolio specializes in both endpoint detection and response (EDR) and application control. Bringing both network and data telemetry to Carbon Black will enable greater visibility and control for our joint customers. Under the new Enterprise Security Group, customers will continue to receive the best service with more dedicated resources and focused support than ever before. What can you expect in the near-term? Let’s take a closer look at innovation, customers, and partners.
InnovationBroadcom’s financial stability empowers Symantec and Carbon Black product portfolios to innovate at a massive scale. Our customers will benefit from access to an enhanced enterprise-grade portfolio and leading technological advancements, with unparalleled service and support.
Initially, we will invest in R&D to improve – and extend the life — of the products our customers are using both on-prem and across hybrid clouds. We also are excited about the complementary nature of both sets of technologies and the combined value they will provide our customers, opening up more choices. For example, Symantec has a data center security product to protect traditional workloads in the data center. Carbon Black has a complementary solution. With access to these two technology sets, defenders will be even better equipped to protect their infrastructures.
On the talent side, we will be making significant investments in engineering. Carbon Black is known for its outstanding, customer-centric engineering capabilities, and we are committed to investing in Carbon Black’s incredible franchise and putting the best talent in the best roles. For example, both Carbon Black and Symantec have existing engineering sites in India. While we see the opportunity to converge them, we do not expect to make headcount reductions in those sites. In fact, we plan to make more investments in India. In addition, we will continue to invest in support and R&D for both brands, retaining key technical and product leaders to ensure continued success today and in the future.
CustomersSymantec and Carbon Black product portfolios will continue to operate in their current states for the immediate future. Our customers can expect to gain access to an extensive and robust enterprise-class portfolio supported by top-tier security experts, intelligence, and continued innovation, all backed by the financial stability of Broadcom.
Looking ahead, we will explore innovative new ways to deliver solutions to our customers. This will involve intensely focusing on the technologies that provide the most value to our customers and partners and invest more resources in those areas so our customers realize even greater value and ROI.
PartnersAt Broadcom, we take a very focused go-to-market approach. As Hock Tan, our CEO, says, “We do what we do best.” By focusing on our core strengths and not trying to be all things to everyone, we open big opportunities for our partners to step in, fill gaps, and profit from them. The addition of the Carbon Black portfolio provides a great opportunity for our partners to drive more revenue, win more customers, and grow. Broadcom will provide the necessary training, support and other resources to ensure our partners’ success with Carbon Black solutions. For examples of our breakthrough approach to building a highly scalable, close-to-the-customer partner ecosystem, look no further than our Global Cybersecurity Aggregator Program (GSAP) and the Expert Advantage Partner Program. Partners in these programs deliver high-value services to customers of all sizes – including our largest enterprise accounts.
Building a strong security futureOver the next few weeks, we will be sharing more details about how today’s announcement will further benefit our customers and partners. In the meantime, we encourage you to visit our online resources to access additional information. As ransomware attacks and other cybersecurity threats continue to rise, you can be rest assured that Carbon Black and Symantec together will provide the mission-critical technologies to defend the most complex, highly regulated organizations.
The post Broadcom brings together two proven portfolios to deliver complete hybrid cloud cybersecurity appeared first on ELE Times.
element14 brings latest Silex Wi-Fi 6 Radio Development Kit from Gateworks to Industrial Developers Worldwide
element14 will be the only distributor to offer this kit for sale at Embedded World 2024.
element14 has announced the availability of Gateworks’ latest Wi-Fi 6 Development Kit – GW11048-5-A.
Gateworks will showcase its cutting-edge technology kit during the Embedded World 2024 exhibition in Nuremberg, Germany, from the 9th to 11th April. element14 has been chosen as the exclusive distributor of the kit, making it the only place where customers can purchase it during the event.
The Wi-Fi 6 Development Kit is designed to facilitate the validation of the Silex SX-SDMAX and SX-PCEAX Wi-Fi 6 radios, providing developers with a seamless out-of-the-box evaluation experience. It delivers ruggedized wireless connectivity for a diverse range of industrial applications, from remote real-time monitoring to predictive maintenance and enhanced logistics.
This latest innovation comes with a pre-loaded Linux system and all the necessary accessories to get started quickly.
Key features include:
- Supports Silex SX-SDMAX and SX-PCEAX Wi-Fi 6 radios (radios not included)
- Includes Venice GW7200 Single Board Computer (SBC) with pre-loaded Linux drivers
- Onboard NXP i.MX8M Mini processor (1.6 GHz quad-core)
- 8GB eMMC flash storage and 1GB LPDDR4 DRAM
- Two Gigabit Ethernet ports and two Mini-PCIe expansion slots
- Multiple connectivity options including MicroSD, Nano SIM, I2C, SPI, and serial ports
- Real-time clock, voltage and temperature monitoring
- Wide input voltage range (8 to 60VDC) with PoE support
- Operates in temperatures ranging from -40°C to +85°C
“We are thrilled to provide Gateworks’ WI-FI 6 development kit to our customers. This cutting-edge technology is a game-changer for those seeking to develop and deploy IoT applications and systems. We look forward to seeing its capabilities showcased at the exhibition and support our customers with all their development needs”, said Romain Soreau, Head of Single Board Computing at element14.
In addition to the Wi-Fi 6 Development Kit, element14 also offers Gateworks Corporation’s complete line of products for industrial applications.
Gateworks will be highlighting their line of railway solutions at Embedded World, aimed at enhancing the efficiency and safety of rail yards, such as rugged Gateworks Single Board Computers (SBCs) combined with a variety of wireless options such as high-precision GNSS Mini-PCIe cards. These solutions enable capabilities such as centimetre-level accuracy in tracking and monitoring critical data, streamlined yard operations and enhanced customer service for rail operators.
The post element14 brings latest Silex Wi-Fi 6 Radio Development Kit from Gateworks to Industrial Developers Worldwide appeared first on ELE Times.
Designers lead advances in CT scanning field
Courtesy: Avnet
When a doctor tells you to get a CT scan, they’re calling on a powerful medical imaging technology for insights only otherwise possible through invasive procedures.
Computed tomography (CT) exploits the penetrating nature of X-rays. A standard X-ray shines a 2D beam of high-energy photons through the subject. How these photons are detected has changed over the years. It was once just photographic film, but today it is more likely to be a digital detector.
Since bone, muscle and fat each absorb X-rays differently, the image captured is effectively the shadow cast by the mix of tissues in the body. Rather than create an image directly from the photons detected, computed tomography processes that captured data to synthesize an image.
In CT scanning, a source illuminates the subject using a fan-shaped beam of X-rays that are picked up by an arc-shaped array of digital detectors. The source and the detector are mounted on a circular gantry, which rotates around the patient, taking scans from multiple angles.
The resulting scans are not directly interpretable as an image. The scans are combined in a computer, creating a more detailed 2D “slice” through the body. Many CT scanners also coordinate the movement of the patient with the gantry’s rotation, creating a sequence of slices through the body that can be processed into a 3D image.
Seeing the advantages of CT in medical imaging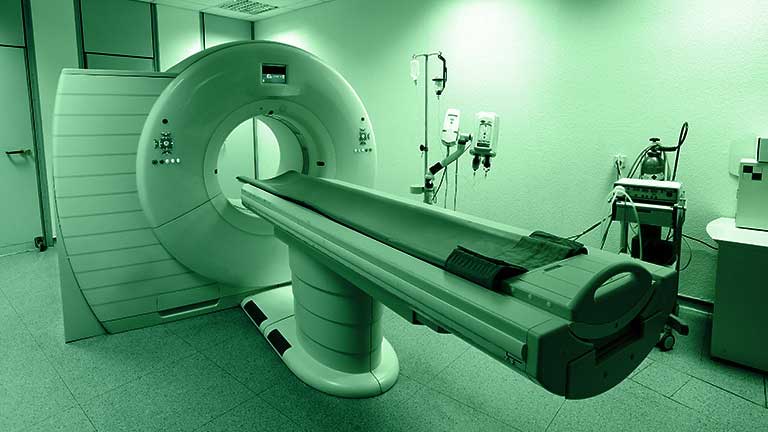
patients.
CT scans can render more detail about internal structures than ordinary X-rays and can present that data in augmented 2D or 3D, making it easier to interpret. They are also relatively fast, which makes them useful for providing insights about injuries to the head, spine, chest, abdomen and pelvis.
The ability to post-process CT images means that they can provide a useful basis for detecting tumors and cancers, their size, location, and how they have spread. They can also reveal internal bleeding and the spread of infection as well as enable doctors to visualize blood vessels, aneurysms and blockages throughout the body.
CT imaging can be used to reduce the invasiveness of some procedures. The ability to differentiate diseased tissue helps surgeons avoid removing healthy tissue unnecessarily. Similarly, the 3D detail possible with CT imaging can provide a useful basis for planning procedures such as biopsies, surgery, implants, and radiation treatment.
The technique can reveal the detailed health of bones and joints, making it easier to understand wear or disease and to diagnose fractures. CT scans can also help track the progress of disease and reveal the effectiveness of treatments such as chemotherapy.
There are challenges associated with CT scanning. They usually involve greater exposure to ionizing radiation than is common with standard X-rays. Patients may also react badly to the contrast agents used to improve the CT scan’s effectiveness.
Navigating the key trade-offs in CT scanner design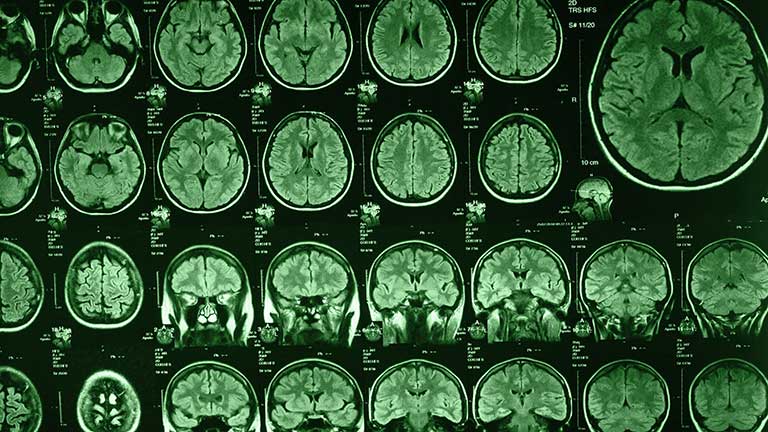
Developers of CT scanners work with two forms of constraints. The first is the paramount nature of combining innovation with patient safety when using ionizing radiation. The second is the tension that can bring for patients and care providers. The availability of new technologies and capabilities must always be met with the best judgment and a conservative attitude.
There are technical trade-offs in CT design too. Perhaps the most important of these is between image quality and radiation dose levels. Higher doses may improve image clarity at the cost of greater exposure. Technologies such as iterative image reconstruction and denoising algorithms based on machine-learning techniques can now replicate some of the image-quality gains of high-dose scans at lower doses.
Trade-offs also exist between the speed of scanning and image resolution. Faster scanning reduces artifacts introduced by patient movements but can result in lower spatial resolutions. Scanning more slowly can deliver higher resolution if the patient is still for long enough.
Designers also need to decide which market niche they want to address. For example, designing a scanner with a large field of view makes it easier to scan large body parts, but requires larger, more expensive detector arrays. Other trade-offs may have to be made between scanner flexibility and specialization, hardware quality and maintenance costs, and software capabilities and reliability.
There are also financial considerations over initial cost and long-term upgradability. Buying a CT scanner means a large upfront outlay and substantial operating costs. Buyers may be prepared to choose a scanner engineered to evolve, rather than a lower-cost machine with a more limited useful lifetime.
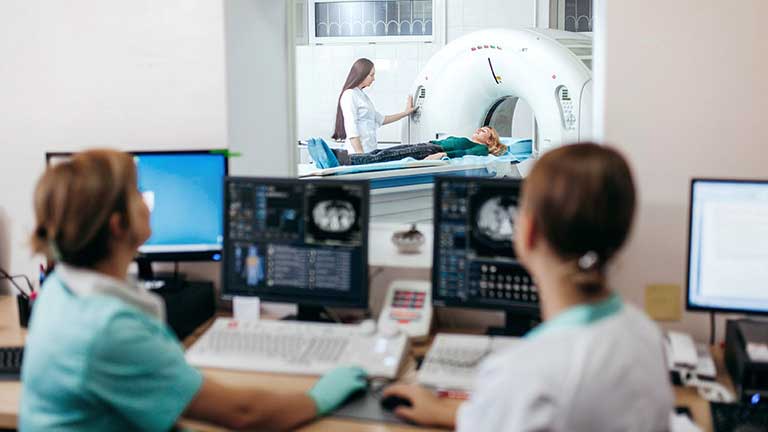
Despite the constraints and trade-offs outlined above, there are many avenues for innovation in CT design. Each detector usually has a scintillator, which emits visible light when it is hit with X-rays, mounted over a digitizing photodetector circuit. A basic CT scanner will have one arc of these detectors, but more sophisticated variants will have multiple arcs so that they can sample multiple “slices” simultaneously. There may be as many as 256 arcs.
To support the high number of detectors, semiconductor companies are engineering 128-channel analog-to-digital converters (ADCs). These ADCs can be mounted in modules to produce 256-channel capabilities. The chips have low-power, low-noise, low-input-current integrators. Simultaneous sample-and-hold circuits ensure that all samples are taken at once. Some ADCs targeting medical applications offer resolutions of up to 24 bits.
Achieving low-dose CT imagingDifferent beam energies can reveal different things about the subject they are illuminating. Radiologists can adjust the beam strength used in the scan to pick out specific details. This is called the spectral CT technique.
Another approach is to use a dual-layer detector, with the top layer absorbing the lower-energy X-ray photons and a lower layer absorbing the higher-energy photons. This technique can reveal more about how the X-rays have been affected by their passage through the subject material.
A further innovation involves single-photon capture detection, in which a semiconductor device is used to directly count each X-ray photon. This gives scope for lower-dose CT imaging, since it does away with potential photon losses in the scintillation process of conventional detectors. It also makes it possible to measure the arrival energy of every photon, again giving greater insights into how it has been affected by passing through the patient.
In dual-source CTs, two source/detector array pairs are mounted on the rotating gantry ring at 90 degrees to each other. This arrangement gives good coverage of the patient while minimizing interference between the sources.
The two sources can run at different energies, which brings the advantages of spectral CT discussed above. They can also acquire a whole slice image more quickly than a single-source scanner, which gives them greater temporal resolution for imaging moving features such as a beating heart. This in turn reduces motion artefacts in the final scan. Faster scans may also be more acceptable to some patients.
Developing high-resolution CT scannersHigh-resolution CT scanners produce very thin slices of less than 1 mm. They use more, smaller detectors, to achieve higher spatial resolutions than standard scanners. The extra resolution makes it easier to detect and characterize small features accurately.
Such scanners usually have sophisticated image-reconstruction algorithms to enhance image quality and detail, which is particularly important for visualizing fine structures and edges. They can also have features such as enhanced X-ray beam management. These techniques give higher contrast images than standard scanners.
CT scanners are enormously valuable for producing insights into patient health without the need for invasive procedures. Their developers can call on rapidly evolving technologies, such as detector electronics and machine-learning techniques, to provide enormous scope for innovation. Responsibility for patient safety means the adoption of new technologies can feel slow.
Fortunately, designers can make a real difference here by exploring the systemic trade-offs involved in the development of novel CT scanners to produce capabilities that are engineered to encourage rapid uptake. For example, designing a detector sampling and digitization circuit with a lower noise floor will enable higher-resolution scans at the same beam energy, or similar resolutions at lower doses.
An FPGA accelerator board may be used to speed up image-processing algorithms, increasing the scanner’s throughput and so cutting the cost of individual scans. Or perhaps there’s a better way to manage power use in the scanner, extending its reliability and so cutting its operating costs.
Avnet recognizes the holistic challenge of developing medical imaging products and has the resources to help OEMs address them.
The post Designers lead advances in CT scanning field appeared first on ELE Times.