Українською
  In English
Feed aggregator
All you need to know about why and how of thermistor!
Meaning and definition of a thermistor
The word ‘thermistor’ is a combination of two words ‘thermal’ and ‘resistor’. The word ‘thermal’ means ‘heat energy’ which is measured as temperature, whereas ‘resistor’ means ‘an equipment that obstructs the flow of electricity, that is, current, both direct and alternating’.
Put simply, a thermistor is a temperature-sensor that measures temperature with respect to change in resistance. It so happens because it is made up of a semi-conducting material that exhibits a ‘precise and large’ change in resistance with respect to a small change in temperature.
Since it is made up of a semi-conducting material, it exhibits resistance intermediate between the resistance of conducting and insulating materials.
Furthermore, the relationship between the variation of a thermistor’s resistance with respect to temperature is dependent upon the ‘resistance-coefficient’ of the semi-conducting material used to make a thermistor. Either it can be positively-related or negatively-related.
Even though both thermistor and resistance temperature detector (RTD) measure temperature with respect to change in electrical resistance, they are different!
This difference is based on the material used to construct them and the temperature range over which they are used. The former uses metallic oxides and operates at fairly low temperature range, whereas the latter uses pure metal and operates at high temperature range.
Components of a thermistor
A thermistor is composed of two wires that are connected to a semiconducting material. All these are pressed into a disk, cylindrical shape, or bead, and then compressed inside an impermeable material, for instance, a glass.
This semi-conducting material is made up of a wide range of materials depending upon the requirement. It is then connected to an ohm-metre. The latter measures the change in resistance of the semi-conducting material.
Although the semi-conducting material of a thermistor is usually made up of either transition metal oxides, such as, oxides of manganese and nickel, or perovskite crystals, such as, strontium titanate and lead (II) titanate, nowadays, it is also made up of cement-based material, conductive polymers and graphene.
Working principle of a thermistor
A thermistor functions based on the movement of electrons or lattice aggregate with respect to change in temperature and the Steinhart–Hart equation.
This equation gives the relationship between resistance of a semi-conducting material and temperature. With this equation, for any specific semi-conducting material, the change in resistance with respect to change in temperature, and vice-versa, can be computed.
Besides, over a temperature range of 200 °C, the error in computation from the Steinhart–Hart equation is less than 0.02 °C. Hence, it yields a highly accurate temperature measurement.
When a thermistor is put at a point where temperature is required to be measured, due to thermal energy at that point, either the valence electrons from the lattice atoms are loosened or the thermal lattice agitations changes. This leads to change in resistance of the semi-conducting material based on its resistance-coefficient. When this change in resistance is measured and then applied in the Steinhart–Hart equation, it yields the temperature at that point.
Types of thermistors
Thermistors are classified based on the basis of the ‘resistance-coefficient’ of the semi-conducting material that makes up a thermistor. They are of the following types-
First, negative temperature coefficient thermistor. In this type, the resistance of its semi-conducting material is negatively related to temperature, that is, the resistance decreases with an increase in temperature.
It so happens because with a rise in temperature, the valence electrons from the lattice atoms are loosened. As these electrons move, they transport electricity more easily. Hence, the resistance decreases.
The material used to make a negative temperature coefficient thermistor is decided based on the temperature range required to be measured. For instance, germanium measures temperature in the range of 1 Kelvin to 100 Kelvin, whereas silicon measures temperature upto 250 K.
On the other hand, the metallic oxides measure temperature in the range of 200 K to 700 K. Hence, to measure higher temperature ranges, thermistors are manufactured using oxides of beryllium, yttrium, zirconium, dysprosium, aluminium, etc.
Second, positive temperature coefficient thermistor. In this type, resistance of its semi-conducting material is positively related to temperature, that is, the resistance increases with an increase in temperature.
It so happens because with rise in temperature, there is an increase in the thermal lattice agitations, particularly those of impurities that are used to dope the semi-conducting material. This obstructs the flow of electricity. Hence, the resistance increases.
A positive temperature coefficient thermistor is of two types-
One, switching positive thermal coefficient thermistors. These thermistors show a non-linear relationship with change in temperature.
As a result, initially, the resistance decreases minutely with a rise in temperature. But, once a critical temperature, known as Curie temperature, is reached, the resistance rises rapidly with rise in temperature. Hence, they are ideally suited for protective applications.
Two, silistor positive thermal coefficient thermistors. These thermistors show a linear relationship with change in temperature. Resultantly, the resistance increases with a rise in temperature.
Among all the thermistors, the negative temperature coefficient thermistors are the most widely used.
Benefits of using a thermistor
There are numerous benefits of using a thermistor. They are as following-
First, they are ideally suited for detecting small changes in temperature. It is so because they have higher sensitivity and shorter response time. In fact, they are the most accurate temperature-sensors.
Second, their cost of production is low. Hence, they can be produced en masse at a low cost.
Third, chemically, they are highly stable and not significantly affected by aging, that is, use over a reckonable period of time.
Limitations of using a thermistor
Besides having numerous benefits, there are few limitations of the use of thermistors. Few of them are as enumerated below-
First, they function over a limited temperature range, mostly between 0°C to 100°C. However, with new advances in technology, the temperature range of functioning of a thermistor has increased. However, due to relatively high cost of production involved in the large-scale production of the latter, most thermistors produced are functional within the stated range.
Second, they work only up to 50°C of the base temperature.
Applications of thermistor
Depending upon the benefits and limitations of the use of thermistors, they are used in as following-
First, since thermistors are ideal for detecting small temperature changes, they are used in an array of temperature-measuring devices. For instance, hair dryers, refrigerators, freezers, toasters, etc., use thermistors for accurate temperature sensing.
Second, they are employed to measure radio-frequency power and radiant power. For instance, infrared and visible light.
Third, they are used in myriad electrical circuits for two purposes. One, to compensate for changes in temperature of the other components. And, two, to detect overload and thus prevent any further flow of electric current in the circuit.
Fourth, they are used in mobile phones to keep a watch on the temperature of different components and relay data to the integrated circuit about it.
Fifth, they are used in microwaves to prevent it from overheating and catching fire.
Sixth, they are used in automobiles for measuring the temperature of different components, oil and coolant.
Seventh, they are used in washing machines to determine the optimum temperature for the operation of washer and dryer.
Eighth, they are used in lithium battery rechargers to stop further charging once the battery is fully charged.
The post All you need to know about why and how of thermistor! appeared first on ELE Times.
first project
![]() | my first project after 1 month of learning.Displaying decimal numbers ( 0 - 3 ) from binary number. Full made of transistors and diodes. [link] [comments] |
Voltage inverter design idea transmogrifies into a 1MHz VFC

It’s interesting, useful, and fun that basic electronic topologies often turn out to have utility in multiple and surprisingly different applications. Figure 1 shows an example of such a circuit. It’s a charge pump voltage inverter circuit originally published in A simple, accurate, and efficient charge pump voltage inverter for $1.
Figure 1 Basic voltage inverter circuit scaled for efficiency at 100 kHz and several milliamps of current output.
Configured thusly for the voltage inverter application, the pump is simple and cheap. It draws only about 1µA per kHz (unloaded) from the 5-V rail.
Wow the engineering world with your unique design: Design Ideas Submission Guide
An interesting variation results if pump capacitor C2 is reduced by several orders of magnitude. This makes the current pumped directly proportional to oscillator frequency: Ipump = 5*C2*Fpump
Starting from that idea, then adding some simple discrete components, our original inverter circuit becomes the core of an inexpensive, fast (1 MHz), low power voltage to frequency converter. Figure 2 shows how.
Figure 2 Modified voltage inverter becomes power thrifty 1MHz VFC.
Input current = Vin/R1 charges C3 which causes transconductance amplifier Q1,Q2 to sink, increasing current from Schmidt trigger oscillator cap C1. This increases U1c oscillator frequency and the current pumped by U1a,b and C2. This is because the pump current has negative polarity (remember we started with a voltage inverter circuit); it completes a feedback loop that continuously balances pump current to equal input current:
Ipump = 5*C2*Fpump = Vin/R1
Fpump = Vin/(5*C2*R1) = Vin/(5*100pF*10,000) = 200kHz*Vin
Q3 provides the ramp reset pulse that initiates each oscillator cycle. R6 limits C2 discharge current to prevent driving U1 pin 1 substrate diodes into conduction, which could steal a fraction of Ipump and thus create nonlinearity. The ratio of R5/R3 is chosen to balance Q2/Q1 collector currents at Vin and Fpump equal zero, thus minimizing Vin zero offset. Consequently, linearity and zero offset errors are less than 1% of full-scale.
However, this leaves open the possibility of unacceptable scale factor error if the +5-logic power rail isn’t accurate enough.
What if we want a precision voltage reference that’s independent of +5 instability? Figure 3 answers that question.
Figure 3 U2 shunt reference stabilizes C2 charge to a +5 independent precision 2.50 V.
Adding the reference does, however, increase parts cost by about half a buck and max power consumption by about half a milliamp. These totals are still rather reasonable prices to pay for accurate and fast conversions. Yes, for a VFC, 10-bit resolution in a millisecond is pretty fast.
Note that R1 can be chosen to implement almost any desired Vin full-scale factor.
Stephen Woodward’s relationship with EDN’s DI column goes back quite a long way. Over 100 submissions have been accepted since his first contribution back in 1974.
Related Content
- A simple, accurate, and efficient charge pump voltage inverter for $1 (in singles)
- Single supply 200kHz VFC with bipolar differential inputs
- New VFC uses flip-flops as high speed, precision analog switches
- Inexpensive VFC features good linearity and dynamic range
- Turn negative regulator “upside-down” to create bipolar supply from single source
The post Voltage inverter design idea transmogrifies into a 1MHz VFC appeared first on EDN.
makeITcircular 2024 content launched – Part of Maker Faire Rome 2024
Applications to MakeITcircular must be in by October 3, 2024 with the top prize of €3000 MakeITcircular is the contest that promotes the Circular Consumption Charter and the values of recycling, reuse and co-design. Shifting from a linear to a “circular economy” model is the key to enabling economic growth that respects material and natural resources and to making […]
The post makeITcircular 2024 content launched – Part of Maker Faire Rome 2024 appeared first on Open Electronics. The author is Boris Landoni
LTE Cat 1bis An option for Low Power Wide Area (LPWA) applications
Courtesy: u-blox
The Internet of Things (IoT) ecosystem todayThe IoT ecosystem is experiencing a revolutionary phase. Most Mobile Network Operators (MNOs) have announced or even executed the switch-off of legacy 2G and 3G cellular technologies, migrating the related frequencies to 4G or 5G networks. As a result, IoT developers must also adopt a new cellular standard.
Although 5G is already under deployment, its use faces several challenges. Besides limitations in global coverage, current 5G solutions don’t meet most IoT requirements: high power consumption, cost, size, and design complexity are some of the drawbacks. 5G RedCap and MMTC are expected to address these issues in the future. For now, 4G LTE is the only cellular technology that provides ubiquitous global coverage and can play this role for at least the next five years.
A bipolar landscapeIn 2016, 3GPP Rel 13 specified new 4G cellular standards for the IoT. The aim was to compete with non-cellular Low Power Wide Area (LPWA) technologies, like SigFox or LoRaWAN. The new cellular standards that 3GPP launched were NB-IoT (aka LTE Cat-NB), LTE-M (aka LTE Cat-M), and LTE Cat 1bis (3GPP Rel 8 had already specified the LTE Cat 1 standard).
Following 3GPP Rel 13, MNOs and chip manufacturers focused on NB-IoT and LTE-M, leaving aside LTE Cat 1bis. This decision was made because, comparing specs, the Cat 1bis pros/cons ratio was less favourable than the other two.
An intense debate arose around the new proposed cellular LPWA technologies for the IoT. One side pushed toward using NB-IoT, while the other toward LTE-M as in the past, countries could independently agree on deployed technology, supported bands, and roaming agreements.
But apart from a few exceptions like IMT-2000’s early rollout in Japan or the initial deployment of CDMA/EV-DO in North America before converging to UMTS/HSPA, the world was under global convergence.
More recently, that convergence was left out of the picture. APAC and most of EMEA chose NB-IoT implementations, while the Americas, Japan, South Korea, and Australia chose LTE-M.
The divergence created a bipolar world. China pushed hard for NB-IoT; indeed, China is the only country where NB-IoT has had a massive deployment. North America, on the other hand, deployed LTE-M quickly. Today, LTE-M dominates most of this market, although some MNOs have added NB-IoT support over the years. EMEA’s case is peculiar. Initially, it opted for NB-IoT. Later, however, most MNOs in Western European countries also added LTE-M support.
While LTE-M characteristics fit most IoT requirements, this is not true for NB-IoT. Even if NB-IoT has better MCL (Maximum Coupling Loss) than LTE-M, its data rate is limited. In addition, the design of NB-IoT infrastructure does not support mobility (handover) and voice.
In the current scenario, we find regions where IoT applications benefit from excellent LTE-M coverage (like North America), whereas in others, it’s partially or entirely absent. When the latter situation happens, NB-IoT cannot satisfy most use cases due to the abovementioned limitations.
In Europe, many IoT applications still connect to legacy 2G (where available). Customers ask for LPWA modules with 2G fallback because LTE-M coverage is unreliable and, in worst cases, absent. NB-IoT capabilities do not fulfill use case demands.
Until recently, LTE Cat 1 was the lowest-cost 4G cellular technology with global coverage. A drawback of LTE Cat 1 is that its chipsets cost much more than those for NB-IoT or LTE-M. Moreover, an LTE Cat 1 design is more complex and requires additional components compared to LTE-M and NB-IoT, which significantly impacts the overall cost.
This situation has led IoT developers and MNOs to search for cost-effective alternatives and thus guarantee LPWA worldwide connectivity, mobility, and roaming for the IoT ecosystem — resulting in a renewed interest in LTE Cat 1bis.
What is Cat 1bis?In a nutshell, LTE Cat 1bis is LTE Cat 1 with a single receive (Rx) antenna. All the other device characteristics, like uplink (UL) / downlink (DL) data rates and protocols, remain the same. On the contrary, standard LTE Cat 1 (3GPP Rel 8) supports Rx diversity and requires two Rx paths.
Rx diversity improves RF reception capabilities, especially at the edge of the cell. But to support Rx diversity, the LTE Cat 1 chipsets require two RF inputs with a sophisticated RF front-end and specific software. To some extent, this is why LTE Cat 1 chipsets cost more than those for LTE-M and NB-IoT, and why LTE Cat 1 applications require more elaborated and extensive PCBs, additional components, and two antennas. All these features result in higher costs: an LTE Cat 1 solution could cost twice as much as its LTE-M counterpart.
Removing the Rx diversity feature from the LTE Cat 1bis standard enables simpler, cost-optimized chipset designs. Developers are taking advantage of this to design simpler, smaller, and cheaper IoT applications than they could with the LTE Cat 1 standard.
Why consider LTE Cat 1bis as an additional option for LPWA?- Comparing LTE-M and LTE Cat 1bis, both can be suitable technology choices for IoT applications, depending on the region and use case. There are several reasons for this:
- Power consumption: Both LTE-M and LTE Cat 1bis support low-power modes like PSM and eDRX. These extend battery life, an important feature for many IoT applications.
- Total solution cost: Both LTE-M and LTE Cat 1bis can cost half as much as an equivalent solution based on LTE Cat 1, representing significant savings.
- 5G compatibility: LTE Cat 1bis is a 4G network technology that connects to the 4G Evolved Packet Core (EPC). In theory, LTE-M and NB-IoT standards can connect to the 5G core. Yet, MNOs have not implemented this functionality, and none have announced plans to do so.
- Data rate/latency: LTE Cat 1bis outperforms LTE-M in terms of latency and data rate, matching the capabilities of LTE Cat 1 with up to 10 Mb/s downlink and 5 MB/s uplink. This often exceeds the requirements of IoT applications. LTE-M has a downlink limit of 375 kb/s and an uplink limit of 1 Mb/s.
To summarize, both LTE Cat 1bis and LTE-M meet the requirements of most IoT use cases previously served by 2G and 3G technologies: medium bandwidth, low power consumption, and low cost, among others.
Why consider LTE Cat 1bis as an LPWA alternative now? What driving factors could lead IoT developers to ponder LTE Cat 1bis as an LPWA option? We must consider the specific use case, price gap, and status of LPWA global deployments to answer these questions. When choosing the proper communication technology, four considerations are at stake. The following is a brief but by no means exhaustive list:
- The amount of transmitted data and the impact on the battery
- Network coverage and availability
- Service lifespan
- Link budget
- Device size
We must consider that IoT applications like video surveillance, alarm systems with video, or eHealth, produce considerable data volume. These use cases could leverage LTE Cat 1bis operators with 20 MHz bandwidth compared to 1.4 MHz for LTE-M.
With more bandwidth, devices can transmit data faster. This translates into less time in the air and, consequently, less battery usage. Depending on the amount of data, faster transmission means less time in the air, resulting in better power efficiency.
Network coverageThe global portability provided by legacy 2G and 3G technologies is ending due to the sunset of cellular standards in many countries. LPWA technologies such as LTE-M and NB-IoT have been deployed without much coordination, creating a peculiar global situation. APAC and most Eastern European countries have only NB-IoT coverage, whereas the Americas, Australia, and a few European countries have both NB-IoT and LTE-M. Although in this latter case, the coverage faces several obstacles.4G LTE is present in most African countries where neither NB-IoT nor LTE-M has been deployed. The exception to the rule is South Africa, where NB-IoT is currently active.
Roaming agreements should also be considered. They are scarce, and there is no guarantee that an IoT device traveling between countries will have network access, even if there is sufficient coverage in both locations.
Consider Italy as an example, although this applies to other Western European countries as well. Italian MNOs, like most European MNOs, initially deployed NB-IoT. But in recent times, Vodafone Italy added LTE-M support (2022). This does not necessarily mean that LTE-M is available everywhere, especially considering that the NB-IoT coverage is not comprehensive. So even if LTE-M is available, the Italian territory lacks full coverage with no certainty when this will be achieved.
Another example is the United States, where LTE-M has been extensively deployed with good coverage across most of the country, resulting in a large installed base of LTE-M IoT devices. Yet, NB-IoT has been less prioritized. Although US MNOs have deployed NB-IoT networks, the installed base is much smaller compared to LTE-M.
LTE Cat 1bis is available wherever there is a 4G LTE network, which is the case in most populated parts of the world. Roaming between 4G networks is largely possible under existing agreements, making LTE Cat 1bis particularly suitable for mobile applications such as telematics and asset tracking. Examples like this could encourage IoT developers to consider LTE Cat 1bis as a viable LPWA option.
Service lifespanCurrently, it is difficult to say which technology, LTE Cat 1bis or LTE-M, will have a longer lifespan. The MNOs have not announced any sunset plans for either technology, and the timing is likely to vary from region to region.
Some markets, like the United States and some APAC countries, may transition to 5G more quickly. This could incentivize network operators to shift from the current 4G spectrum to 5G. Factors to consider may include the number of legacy devices on their networks, the bandwidth each technology consumes, and which bands will be prioritized for the transition. Other markets, however, will move much more slowly and may keep both technologies alive well into the late 2030s.
Link budgetLTE-M has a Maximum Coupling Loss (MCL) of -154 dBm compared to -149 dBm for LTE Cat 1. LTE Cat 1bis experiences an additional 3-4 dB loss compared to Cat 1 due to the absence of the Rx diversity antenna. This means that the MCL of LTE Cat 1bis is 8-9 dBm worse than LTE-M. The higher MCL of LTE-M ensures better connectivity in challenging signal conditions, such as harsh urban environments, garages, or at the cell edge. However, LTE-M’s deeper signal penetration is partially offset by its lower cell density compared to standard 4G LTE.
Device sizeDevelopers find it hard to address some use cases, even considering the current miniaturization of electronic components. LTE Cat 1 ticks many boxes for use cases like wearables that require small size and medium data rates, offering advantages in terms of bandwidth, coverage, and power consumption. Still, miniaturization has been challenging due to the need for a dual-antenna design.
The fact that designers aim for small solutions forces them to find the equilibrium between performance and size. Consequently, many current small form factor LTE Cat 1 designs omit Rx Diversity and do not include a second antenna.
With its simplified antenna, shorter parts list, and more affordability (compared to LTE Cat 1), LTE Cat 1bis can also replace LTE Cat 1 in many use cases.
ConclusionBoth LTE Cat 1bis and LTE-M are suitable technology choices for LPWA applications. So before making a choice, one must consider technology characteristics and regional deployment. One may be a better candidate than the other, or maybe both could be considered. u-blox can assist with this type of selection, depending on specific use cases and MNOs.
u-blox offers a broad portfolio, including LTE-M, LTE Cat 1, and LTE Cat 1bis modules. u-blox established early market leadership in LTE-M with the first certified LTE-M module series, SARA-R4, which also offers 2G fallback. u-blox also developed its own LTE-M chipset, the UBX-R5, which was used as the basis for the u-blox SARA-R5 module series. Both series have been further miniaturized into the LEXI-R4 and LEXI-R5 form factors.
For LTE Cat 1bis, u-blox offers the LENA-R8 and LEXI-R10 series. LENA-R8 is also available as a combo: LTE Cat 1bis + GNSS variant. The GNSS core is the new u-blox M10 GNSS platform. The LENA-R8 combo (LENA-R8M10) has two power supplies, providing customers with excellent power management flexibility, thus optimizing the overall performance. LEXI-R10 is the world’s smallest LTE Cat 1bis module supporting Wi-Fi scanning and CellLocate for indoor positioning. The u-blox LARA-R6 LTE Cat 1 module supports full Rx diversity and is best suited for high-performance applications.
The post LTE Cat 1bis An option for Low Power Wide Area (LPWA) applications appeared first on ELE Times.
КПІшниця презентувала свій стартап у Римі
Цьогорічна освітня програма «Насіння для майбутнього», що проходила у Римі з 1 до 5 липня, об’єднала 150 студентів із 23 країн світу.
Intriguing Stereotypes: A Double-Edged Sword
In the tapestry of modern organizations, diversity is not just a buzzword; it’s a critical component for innovation, growth, and sustainability. Yet, despite the strides made in promoting inclusivity, stereotypes continue to be a pervasive force, subtly influencing interactions and decisions in the workplace.
A stereotype is an overgeneralized belief about a particular category of people. It’s human nature to categorize information to understand the world around us. However, when these categorizations become rigid and oversimplified, they lead to stereotyping. From the moment we introduce ourselves, we provide cues—our age, gender, attire—that can trigger stereotypical judgments. These initial impressions, while seemingly innocuous, can have profound implications on how we are perceived and, in turn, how we perceive ourselves.
The Stereotype Threat in ActionThe concept of stereotype threat arises when individuals fear being evaluated through the lens of a negative stereotype associated with their group. This fear can be paralyzing, leading to a self-fulfilling prophecy where the anxiety of confirming the stereotype actually causes underperformance. The Indian film “12th Fail” poignantly illustrates this phenomenon. Manoj, a UPSC interviewee, confronts a panel biased towards English-speaking candidates. Despite feeling the pressure of this stereotype, Manoj demonstrates that language is merely a tool for communication, not a measure of one’s knowledge or capability.
The Irony of Self-StereotypingIronically, the fear of stereotype threat can lead individuals to inadvertently reinforce the very stereotypes they wish to dispel. Consider an older job applicant who, aware of the age bias, overemphasizes their experience or rambles about aging, thereby unintentionally affirming the stereotype of being out-of-touch or verbose.
Implications and SolutionsThe repercussions of stereotype threat extend beyond individual experiences, affecting organizational dynamics. It can result in employee disengagement, poor job attitudes, and ultimately, a decline in performance. To combat this, organizations must foster an environment where diversity is celebrated, and the value of each employee is recognized.
Strategies for Organizations- Increase Awareness:
- Develop Inclusive Policies.
- Adopt Transparent Regulations.
- Celebrate Individual Contributions.
In conclusion, while stereotypes may be an ingrained aspect of human cognition, organizations have the power and responsibility to create spaces where diversity is not just accepted but embraced. By actively working to reduce stereotype threats, organizations can unlock the full potential of their diverse workforce, fostering a culture of inclusivity and respect that propels them towards greater heights.
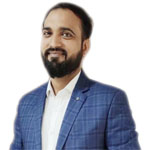
The post Intriguing Stereotypes: A Double-Edged Sword appeared first on ELE Times.
10 Major Thermocouple Manufacturers in India in 2024
In 2023, India’s thermocouple market was worth US$ 778.32 million. The future growth prospect is optimistic. It is expected to grow at a rate of 3.5% between 2024 to 2032. As a result, by 2029, it is expected to have a market worth US$ 926.24 million.
The main reason behind this growth projection is the increase in aggregate demand. It is witnessing a rise due to the following reasons:
First, spurt in demand from different sections of the Indian economy- automobile, steel, manufacturing, e-vehicle, aerospace, oil and gas, power generation industry, a wide array of Internet of Things devices, petrochemicals, mining, etc.
Second, enabling initiatives from the government.
The 10 major thermocouple manufacturers in India are as follows:
- Tempsens Instruments (India) Pvt. Ltd.
Tempsens Instruments (India) Pvt. Ltd. is a subsidiary of the Pyrotech Group. This group was established in 1976 at Udaipur, Rajasthan, whereas Tempsens Instruments (India) Pvt. Ltd. commenced its operations from 1987. Its production facilities are in India, Germany and Indonesia. Its network of distributors operates in 20 countries.
- Honeywell International India Private Limited (HIIPL)
It is the Indian arm of Honeywell International, Inc. based out of the USA. It leverages 100 years of experience of Honeywell International, Inc. In India, it operates three state-of-the-art manufacturing facilities and four global centres of excellence for technology development and innovation.
- ABB India Ltd.
It was incorporated in Mumbai in 1949. It is a subsidiary of the Swedish-Swiss firm ABB Ltd. Its excellence is grounded from the past experience of more than 130 years of the parent firm.
- General Instruments Consortium
It was established in 1966. It operates four state-of-the-art manufacturing sites in India. It produces a wide array of thermocouples.
- S.R.I Electronics
It is based out of Bengaluru, Karnataka. It operates manufacturing plants in Hyderabad, Chennai, Salem, Coimbatore and Surat. Its distribution network is present in all industrial hubs of India.
- RS Components & Controls (India) Ltd.
It is based out of Noida, Uttar Pradesh. It commenced its operations in 1994. It is commonly called RS India because it is a subsidiary of the RS Group plc, London, United Kingdom.
- Sense Thermo Instruments India Pvt. Ltd.
It is based out of Pune, Maharashtra. It produces a wide range of thermocouples. They are used in a variety of industrial applications. However, its speciality is producing thermocouples used in the power sector. For instance, thermocouples used in the blast furnace, gas carburizing, sponge iron plant, hearth furnace, etc.
- Thermo Electric India Pvt. Ltd.
It is based out of Manesar, Haryana. It is a subsidiary of the Thermo Electric Company, Inc., USA. It produces highly reliable thermocouples for a variety of industrial appliances.
- Heatcon Sensors Pvt. Ltd.
It is based out of Bengaluru, Karnataka. It produces a wide range of high-performance thermocouples ranging from base metal thermocouples to noble metal thermocouples. Besides, it also produces multi-point thermocouples.
- Precision Mass Products Pvt. Ltd.
It is based out of Chhatral, Gandhinagar, Gujarat. It was founded in 1967. It produces TCH and TCW types of thermocouples.
The post 10 Major Thermocouple Manufacturers in India in 2024 appeared first on ELE Times.
EEVblog 1630 - Solar Panels for Water Pre-Heating?
ASDC Partners’ Forum 2024: Driving Unity and Innovation in the Automotive Industry
Automotive Skills Development Council (ASDC) successfully hosted the ASDC Partners’ Forum on July 12, 2024, at the Indian Habitat Centre, New Delhi. This significant event brought together industry leaders, experts, and stakeholders to discuss the future of the automotive sector. The theme for this year, “Collaborate, Empower, Accelerate, Achieve,” emphasized ASDC’s dedication to fostering unity and empowerment within the industry for collective success. Dr Neetu Bhagat, Deputy Director of AICTE, Dr Suhas Deshmukh, Director of NCVET, and Dr Rodney Reviere, Cluster Coordinator at GIZ India, attended as Guest of Honour and emphasized the crucial role of skills development in advancing industry progress. ASDC’s Vice President, Vinkesh Gulati, and CEO, Arindam Lahiri, also shared their perspectives.
ASDC Partners’ Forum is a cornerstone event for ASDC and the broader automotive industry. It provides a vital platform for addressing current challenges, exploring opportunities, and formulating strategies to advance the sector. The theme this year reflects ASDC’s ongoing efforts to unite stakeholders and promote empowerment, aiming to drive substantial growth and development. The discussions and outcomes from this forum are expected to have a lasting impact on the industry, fostering innovation and collaboration.
Mr. FR Singhvi, President of ASDC, highlighted the importance of the event, stating, “This annual partner’s forum is crucial for bringing us together to achieve our common goals. By working together and sharing ideas, we can help each other tackle challenges and find new opportunities for growth. ASDC is dedicated to promoting skill development initiatives that improve the abilities and expertise of our youth, ensuring they are well-prepared for their careers. Our recent collaboration with the Central Board of Secondary Education (CBSE) to launch the National Automobile Olympiad (NAO) 2024, with over 850 schools already registered, further underscores our commitment. Such initiatives not only engage and inspire students across India but also highlight the significant impact we can make through collective efforts like this forum.”
A highlight of this year’s forum was the announcement of ASDC’s collaboration with BYD to launch the annual BYD Innovate-a-thon competition. This competition aims to unite students across disciplines in the Electric Vehicle Domain, fostering creativity, teamwork, innovation, sustainability, and excellence. At the ASDC Partners’ Forum, BYD officially launched this initiative with an MOU exchange and a presentation by Mr. Rajeev Chauhan and Ms. Shivani Chaudhary, who will serve as the spokespersons for BYD.
The annual BYD and ASDC Innovate-a-thon unites students across disciplines in the Electric Vehicle Domain, fostering creativity, teamwork, innovation, sustainability, and excellence. Fuel Your Passion for Electric Vehicles with the BYD Innovate-a-thon 2024. The competition will be conducted in three rounds, with the first two rounds held online and the final round held offline.
Compete, Innovate, Win! Register Now! The registration deadline is August 17, 2024. Participants will have the chance to visit BYD Headquarters in Shenzhen and win cash prizes.
Looking ahead, ASDC is committed to continuing its mission of fostering growth and development within the automotive industry. The insights and connections made during this year’s forum will drive new initiatives and partnerships. ASDC aims to empower its stakeholders, promote innovation, and accelerate the industry’s growth. The organization remains dedicated to creating a skilled workforce ready to meet the evolving demands of the sector, ensuring a bright future for all partners and stakeholders.
The post ASDC Partners’ Forum 2024: Driving Unity and Innovation in the Automotive Industry appeared first on ELE Times.
Metcal Introduces BVX-250 Fume Extraction System and HTC 910 Hot Air Rework System
Metcal, a leader in benchtop soldering and fume extraction systems, announces the release of their new BVX-250 Fume Extraction System. The 2-port portable fume extractor offers HEPA filtration of 99.97% efficiency with a significant performance increase over previous models. The system also offers new innovations for changing the filters, exhausting the air, and communicating with the operator, improving ease of use and the user experience.
The BVX-250 has a new patented front-facing main filter and simple-to-use pre-filter drawer, which allow the user to change the filters quickly and easily, without having to remove the top or manipulate the arms of the system. Each filter can now be changed in less than 30 seconds, a major improvement over comparable systems on the market.
The BVX-250 also offers a patented removable 360˚-rotating exhaust diverter which allows users to configure the exhaust direction away from the operator or install the unit directly into ventilation systems. “Since these systems are positioned in many different locations, allowing the exhaust to be customized offers a much-improved user experience,” said Can La, Product Director of Metcal.
With the release of the BVX-250, Metcal has improved communication between the fume extractor and the operator. New features, such as a large LED status ring, and the ability to directly connect the fume extractor to Metcal CV and GT soldering systems, allow for easy-to-view filter notifications from either the fume extractor or the soldering system.
- Easy 30-Second Filter Changes
- LED Status Ring
- 2x the Suction of Previous Models
- 99.97% Efficiency HEPA 13 Filter
- Optional Deep Bed Gas Filter (FG-BVX250, sold separately)
- Quiet Operation
- Three Power Levels
- 360-degree Rotating/Removable Air Diverter
- Communicates with Metcal CV & GT Series Soldering Systems
- New Circular & Rectangular Nozzles with Nozzle Filters are available
- Compatible with previous BVX & Omniflex Arms and Hoses
- Small Footprint for Under or On the Benchtop
- Remote Switch Included
Rework is a necessary part of any soldering operation. Whether machines or humans are assembling the printed circuit boards, inconsistencies happen. When a component is misplaced, misaligned, improperly soldered, or needs to be replaced, typically a soldering iron will be the tool of choice. But for many operators and applications, a solid hot air tool provides additional rework capabilities.
Some of the main concerns for hot air tools is the perceived lack of process control, slower productivity, and safety with hot air. So, how can we alleviate these worries? With the right rework system. Rework situations vary, so it is essential your hot air tool includes precise and simple temperature and flow rate control.
Many hot air systems on the market are not intuitive to use. The menu structures are confusing, the displays are limited, and they are simply not plug-in-play. A system that is not intuitive for the operator is a system that is going to regularly bog down throughput.
When comparing systems, be sure to investigate exactly how the hot air unit operates. Most system manufacturers have demos and how-to videos on YouTube. Watch them to see exactly how each unit is configured. You may be surprised by how few are user-friendly.
Designed with ease of use in mind, Metcal’s new HCT-910 Hot Air Rework System features a new “on-the-fly” manual mode, which allows for fast temperature and airflow adjustments with a single knob (Figure 1). Easily toggle between temperature settings and air flow rate, turn the knob to the desired setting, and push the knob to set.
Enhance Operator Safety with Clear System Status Indicators
KEY FEATURES:
- Status indicator lights on the hand-piece
- Easy to configure and monitor
- Intuitive user interface with convenient presets and admin lockout
- Accurate temperature, up to 600 °C (1112 °F)
- Fast time-to-temperature
- Airflow from 5 – 120 l/m (4.24 cfm)
- Tool-less nozzle/bayonet design
- Replaceable heater
ADDITIONAL FEATURES:
- Clearly designed digital screen for temperature and flow rate display
- System presets and password-protected admin mode
- Multi-language (English, Spanish, German, French, Chinese)
- Hand-piece holder mountable on either side of the unit
For HCT-910 Hot Air Rework System technical data, user guide, how-to videos, and more, go to Metcal.com.
The post Metcal Introduces BVX-250 Fume Extraction System and HTC 910 Hot Air Rework System appeared first on ELE Times.
Weekly discussion, complaint, and rant thread
Open to anything, including discussions, complaints, and rants.
Sub rules do not apply, so don't bother reporting incivility, off-topic, or spam.
Reddit-wide rules do apply.
To see the newest posts, sort the comments by "new" (instead of "best" or "top").
[link] [comments]
EEVblog 1629 - Dumpster Find: A3 Colour Photocopier!
My first ever DIY remote controlled robot!
![]() | submitted by /u/literallybateman [link] [comments] |
Electrode Loading Analysis 2.0: Innovative In-Line Metrology Solution for Battery Production
Thermo Fisher Scientific has launched a state-of-the-art in-line metrology solution for battery manufacturing, providing unmatched electrode mass loading measurement and coating quality assurance. The LInspector Edge In-line Mass Profilometer is designed to revolutionize electrode coating QA by measuring 100% of a coated electrode in real time, without scanning or moving parts. This advanced tool simultaneously gathers spatial information and coating weight data, providing full-width basis weight and profile uniformity analysis with unprecedented precision. It captures data at full production speeds, producing high-resolution images rather than spot measurements typical of conventional gauges. This results in 1000 times more coating profile weight data, enabling the detection of non-uniformities that other technologies might miss. This innovation offers battery manufacturers an exceptional foundation for accurate process control and the highest levels of quality and performance assurance.
Key Features- 100% Electrode Mass Loading Inspection: The LInspector Edge In-line Mass Profilometer allows for real-time inspection of 100% of the coated electrode at high production speeds, providing 1000 times more mass loading uniformity data than traditional scanning gauges. This data helps detect small defects early in the process, enabling effective remedial actions that reduce scrap rates, decrease time to market, and improve overall product performance.
- Advanced Process Control: Electrode coating weight and profile uniformity are critical for maintaining the quality and safety of lithium-ion batteries. The LInspector Edge delivers a complete coating profile every 3 millimeters, offering the fastest measurement feedback for quicker, better-informed, and more accurate control decisions. This capability enhances process efficiency, reduces scrap, and increases throughput during start-up and product transitions.
- Research and Development: For battery R&D, achieving precise electrode coating weight targets is essential for optimal performance and safety. The LInspector Edge provides comprehensive data on electrode coating uniformity, aiding in the optimization of coating materials and equipment controls, which in turn enhances process development, quality, and yield.
- Pilot Scale Manufacturing: In pilot scale production, understanding mass loading distribution is critical for improving product quality and performance. The LInspector Edge accelerates development and improves yields by offering real-time, detailed data on 100% of the coated electrode, facilitating the scale-up of next-generation materials and higher-performance batteries.
- Mass Production: As demand for lithium-ion batteries rises, the challenge is to increase throughput while reducing scrap. The LInspector Edge ensures coating uniformity and faster defect detection, providing comprehensive traceability and high-resolution data for optimized production yield and quality assurance.
Ensuring the quality of battery components throughout the manufacturing process is vital. The LInspector Edge enables complete traceability by providing high-resolution images and data on coating uniformity for the entire production run. This visibility allows manufacturers to identify and address production issues proactively, optimizing the manufacturing process for maximum efficiency.
Empowering SoftwareThe Thermo Scientific LInspector Edge Pro Software enhances the functionality of the LInspector Edge In-line Mass Profilometer by offering real-time mass loading data analysis, high-resolution heat map displays, and comprehensive profile visualizations. Its user-friendly interface allows operators to control the analyzer and process data effectively, making quick adjustments to improve product uniformity and detect defects early.
The software features include:
- Real-Time Mass Loading Data Analysis: Displays multiple visualizations representing the mass loading levels of the entire electrode surface, allowing for quick process adjustments and improved product uniformity.
- High-Resolution Heat Map: Offers a graphical representation of mass loading results, enabling operators to quickly identify areas of concern and adjust the color scale to highlight specific features in the electrode coating.
- CD and MD Profile Displays: Provide detailed cross-section uniformity data and trend graphs for monitoring changes in average weight over time and analyzing key areas of the coating.
- Stripe Profile Display: Gives an overview of each stripe in real-time, highlighting defective areas and displaying key statistics to facilitate faster process control decisions.
By integrating the LInspector Edge In-line Mass Profilometer and LInspector Edge Pro Software, battery manufacturers can achieve higher levels of quality, efficiency, and confidence in their electrode coatings, ensuring the production of safe and reliable batteries.
The post Electrode Loading Analysis 2.0: Innovative In-Line Metrology Solution for Battery Production appeared first on ELE Times.
Advancing Additive Manufacturing: HP’s Innovations in Metal Jet and Jet Fusion 3D Printing
HP has introduced significant advancements to its HP Metal Jet and HP Jet Fusion 3D Printing printers, aimed at enhancing capabilities, introducing new materials, and forging strategic partnerships to propel additive manufacturing forward. The latest improvements to the HP Metal Jet S100 Printing Solution include an expanded build height of up to 170 mm, facilitating the production of larger parts and increasing the volume of parts per build cycle. This innovation leverages Metal Injection Molding (MIM) powders to achieve economies of scale, thereby reducing operational and material costs for large-scale production compared to other 3D printing technologies. With enhanced operational efficiency in printing process times, the HP Metal Jet S100 Printing Solution continues to advance towards achieving optimal industrial Overall Equipment Effectiveness (OEE).
In conjunction with the Rapid + TCT show, HP has announced several enhancements:
- Enhanced Capabilities: The HP Metal Jet S100 Printing Solution now supports increased build height and cost-effective production with new materials such as IndoMIM M2 Tool Steel and Osprey 316L.
- Launch of HP Metal Jet Production Service: This new service platform includes an adoption centre in the US and a global network of contract manufacturers, aiming to democratize 3D metal printing by addressing common barriers such as cost, time, and complexity.
- Strategic Software Updates and Partnerships: HP has bolstered its HP 3D Process Development software by incorporating advanced features such as Multi-level build. Collaborations with industry pioneers including Autodesk, Altair, CoreTechnologie, and Dyndrite have further enriched the integration of cutting-edge design and production tools. The partnership also includes the launch of the HP 3D Texture Visualizer by Leopoly, enhancing the visualization and application of digital textures on CAD geometry in real-time.
Moreover, HP has extended its material capabilities and introduced a new print mode for the HP Jet Fusion 5600 Series 3D Printing Solution. This includes compatibility with HP 3D High Reusability (HR) PA 12 W, HP 3D HR PA 12 S enabled by Arkema, and BASF Ultrasint TPU01 materials, alongside the newly introduced ESD print mode to address specific customer needs and expand production capabilities. These advancements aim to significantly reduce Total Cost of Ownership (TCO) through efficient material usage and enhanced service programs, catering to a diverse range of industrial sectors.
HP’s collaboration with Indo-MIM and Sandvik has resulted in the introduction of new materials like IndoMIM M2 Tool Steel and Sandvik’s Osprey 316L. These materials are designed for applications requiring high-strength mold inserts, cutting tools, improved corrosion resistance, and enhanced processability in industries such as medical, automotive, and industrial manufacturing. Rigorous qualification processes ensure their reliability and accessibility for additive manufacturing applications.
To further support accessibility and adoption, HP has launched the HP Metal Jet Production Service, accessible via a dedicated website. This service offers comprehensive production services, allowing customers to submit queries, request sample parts, and seamlessly transition from prototype to production. The Metal Jet Adoption Center in Corvallis, Oregon, provides end-to-end application qualifications, including benchmarking, sample part testing, and process optimization, supported by HP’s global network of Contract Manufacturers.
In addition to material advancements, HP has strengthened its strategic relationships with leading software companies. Collaborations with Autodesk, Altair, CoreTechnologie, and Dyndrite aim to integrate advanced design and production tools, enhance material information accessibility, and facilitate seamless workflows between design and production processes. These partnerships empower customers to realize innovative applications and streamline additive manufacturing operations.
For HP’s polymer and metal printing customers, the collaboration with Dyndrite supports the entire HP 3D printing ecosystem, enabling users to utilize Dyndrite build manager software for creating and submitting print jobs. HP’s Multi-level build feature within the process development allows for precise adjustment of printing parameters across multiple levels within a single build, enhancing flexibility and control.
Furthermore, HP has introduced the HP 3D Texture Visualizer, developed by Leopoly, to enhance the design process by visualizing digital 3D textures on CAD geometry in real-time. This tool supports the import of custom textures and enables users to adjust parameters like scaling, depth, and resolution to achieve desired visual effects, expanding creative possibilities in 3D design and production.
In conclusion, HP’s advancements in both metal and polymer 3D printing platforms, coupled with strategic partnerships and software innovations, underscore its commitment to driving additive manufacturing forward. These developments aim to enhance production efficiency, reduce costs, and broaden application possibilities across diverse industries, positioning HP as a leader in the evolving landscape of additive manufacturing technologies.
The post Advancing Additive Manufacturing: HP’s Innovations in Metal Jet and Jet Fusion 3D Printing appeared first on ELE Times.
Facilitating Next-Gen Edge AI Computing with FPGAs
In the realm of next-generation Edge AI computing, FPGAs play a pivotal role by offering engineers a blend of low power consumption, scalable performance, and unmatched flexibility. These attributes provide high-performance options that cater to the evolving landscape of AI designs.
Lattice Semiconductor’s MachX05-NX exemplifies this trend, integrating robust security and I/O capabilities crucial for managing and controlling Edge AI applications effectively. As the adoption of next-generation AI at the Edge accelerates across modern enterprises, investments in generative AI are projected to soar from $16 billion in 2023 to over $143 billion within the next three years, highlighting Edge AI’s pivotal role in future automation strategies.
For developers navigating this dynamic landscape, the demand for agile solutions is intensifying, driven by the need for enhanced efficiency in Edge AI computing. FPGAs emerge as a solution of choice, empowering system designers to streamline development cycles and deploy AI models swiftly across diverse applications—from smart homes and factories to intelligent automotive environments.
Ultra-Low Power and Scalable PerformanceEfficient power management is critical for always-on Edge AI devices, where FPGAs deliver high performance with minimal power consumption compared to conventional processors. Their reconfigurable architecture and parallel processing capabilities enable real-time AI inference with low latency and energy efficiency. Unlike traditional CPUs, which process data in batches, FPGAs handle multiple data streams simultaneously, enhancing their suitability for smart cameras and sensor-driven applications that demand rapid data analysis.
In autonomous vehicles, for instance, FPGAs bolster situational awareness by accelerating AI computations for tasks such as object detection and path planning, crucial for real-time decision-making.
Enhanced Flexibility and ProgrammabilityFlexibility and programmability are indispensable for Edge AI applications in the fast-evolving AI landscape. FPGAs offer unparalleled flexibility, allowing devices to be retrofitted or modified post-deployment to align with evolving performance needs, safety requirements, and system upgrades. This capability enhances operational agility and safeguards against customer dissatisfaction and regulatory risks.
Unlike fixed-function processors, FPGAs can be tailored to perform specific AI tasks, optimizing performance for both individual tasks and overall application efficiency. This adaptability makes them an ideal choice for developers seeking to fine-tune hardware to meet the precise demands of Edge AI applications.
Robust Security and PrivacyFPGAs significantly bolster cyber resilience and data privacy in Edge AI environments. By integrating FPGAs into Edge AI infrastructure, developers enhance data security through built-in features like encryption and authentication, mitigating potential cyber risks. Processing data locally on FPGAs reduces the need for extensive data transmission to the cloud, minimizing exposure to security vulnerabilities associated with cloud-based data exchanges.
Moreover, FPGAs serve as hardware roots of trust (HRoT), ensuring data integrity and bolstering overall system security. This inherent security, coupled with their adaptive nature, positions FPGAs as a robust security engine for diverse Edge AI applications, safeguarding sensitive information against unauthorized access.
Driving Innovation in Edge AIThe coexistence of FPGAs and Edge AI computing is catalyzing a new wave of innovation. As Edge AI applications evolve to demand faster processing and greater complexity, FPGAs are poised to lead the charge. Their combination of flexibility, efficiency, and security makes them a preferred choice for developers shaping the future of intelligent Edge devices.
FPGAs are not merely keeping pace with the growth of Edge AI—they are pivotal enablers of its expansion. Looking ahead, their unique capabilities will empower developers to create resilient, high-performance Edge AI solutions that redefine interactions with our increasingly interconnected world.
The post Facilitating Next-Gen Edge AI Computing with FPGAs appeared first on ELE Times.
Innoscience claims victory over EPC’s ’508 patent in ITC’s initial decision
VNT Unveils India’s First 98% Efficient Rectifier
VNT is excited to announce the launch of India’s first 98% efficient rectifier, a revolutionary advancement in the telecom industry. This breakthrough reinforces VNT’s leadership in developing sustainable and energy-efficient solutions.
The new Green DC Ultra High Efficiency 98% Rectifier operates with a voltage range of 85-305 VAC and can withstand temperatures range up to 75 degrees Celsius and humidity levels up to 95%. This innovation is a significant step towards achieving net-zero targets, offering unmatched efficiency, the fastest return on investment, reduced heating, and adaptability to various environmental and electrical conditions.
Telecom operators, who consume 1-2% of the world’s total electricity annually, must reduce carbon emissions from energy usage to meet net-zero goals.
Dr. Vikas Almadi, Chairman & Managing Director, and Mr. Rahul Sharma, CEO of VNT, highlighted at the launch: “In 2021, VNT set a new benchmark with its 97% efficient rectifier, surpassing the market standard of 95%. This innovation allowed telecom operators to optimize electricity usage and significantly cut energy costs. Now, with the 98% efficient rectifier, VNT is pushing efficiency even further.”
Investing in VNT’s 98% efficient rectifier provides a swift route to achieving net-zero targets while offering substantial long-term energy savings. VNT invites telecom companies to embrace this cutting-edge technology and lead the charge towards a more sustainable and connected future.
The post VNT Unveils India’s First 98% Efficient Rectifier appeared first on ELE Times.
Keysight Joins the AI-RAN Alliance to Advance AI Innovations in Mobile Networks
- Joins new consortium focused on integrating artificial intelligence advancing into wireless communications technology to enhance radio access networks
- Brings measurement science expertise to artificial intelligence research areas such as improving spectral efficiency and optimizing radio access network performance
Keysight Technologies, Inc. (NYSE: KEYS) has joined the AI-RAN Alliance to advance the use of artificial intelligence (AI) technology and innovations in radio access networks (RAN). Established in early 2024, the alliance is a collaborative initiative bringing together technology, industry, and academic institutions to integrate AI into cellular technology to enhance RAN performance and mobile network capabilities.
AI is a powerful tool with the potential to revolutionize wireless communications networks. The natural complexity of its architectures and operations make RAN an ideal application for AI in order to drive more optimal, reliable, and efficient mobile networks. In response to this opportunity, the AI-RAN Alliance wants to find ways to enhance mobile network efficiency, reduce power consumption, and retrofit existing infrastructure that will unlock new economic opportunities, facilitated by 5G and 6G. The alliance will do this with RAN-related AI research in the areas of improving spectral efficiency, using architectures more effectively, generating new AI-driven revenue opportunities, and deploying AI at the network edge to offer new services and increase operational efficiency.
Keysight understands the transformative power of AI and the role it will play in shaping the way future communications systems will be built and need to be tested. The company is active in a variety of early research projects ranging from optimizing wireless performance to advancing AI learning in wireless communications testing. Keysight is also part of NIST’s AI Safety Consortium and is engaged in multiple standards and research initiatives related to the nascent field of validation of AI systems.
Ardavan Tehrani, Samsung Research, AI-RAN Alliance Board Director, said: “The AI-RAN Alliance is excited to welcome Keysight as a new member. As the wireless industry matures from AI model development to deployment, there is a need for rigorous testing of these models to ensure consistent and expected performance and reliability of AI models. The domain knowledge Keysight brings in these areas will help further the alliance’s mission of bringing AI technology to the RAN.”
Giampaolo Tardioli, Vice President, 6G and Next Generation Technology at Keysight, said: “With our deep expertise in simulation, modeling, and measurement science, Keysight will provide valuable solutions to accelerate the deployment of AI in the RAN and to improve accuracy and trustworthiness of the models of AI in the RAN. Joining the AI-RAN Alliance is a natural choice and another proof point of Keysight’s commitment in enabling innovation across the communication and computing industries. We look forward to collaborating with the other alliance members to help fully realize the potential of AI for wireless systems.”
The post Keysight Joins the AI-RAN Alliance to Advance AI Innovations in Mobile Networks appeared first on ELE Times.
Pages
