Українською
  In English
Новини світу мікро- та наноелектроніки
PC-based scopes gain 10Base-T1S decoder

All PicoScope oscilloscopes from Pico Technology now include a serial decoder for the 10Base-T1S automotive Ethernet standard. This brings the total number of serial protocol decoders available with the free PicoScope 7 software to 40. The software is compatible with all current PicoScope models, as well as legacy models marketed in the past 7 years or longer.
PicoScope 7 software is compatible with Windows, macOS, and Linux, offering a comprehensive suite of automotive decoders such as CAN, CAN XL, FlexRay, LIN, and now 10Base-T1S. In addition to these, the automotive version of PicoScope 7 introduces support for new vehicle and powertrain types and improved guided tests with waveform library linking.
Pico’s noise, vibration, and harshness (NVH) diagnostics application, PicoDiagnostics NVH, now supports the worldwide harmonized on-board diagnostics (WWH-OBD) protocol. Complementing the already-supported J1939 communication protocol, the app now provides an additional means to acquire speed information from heavy-duty and off-highway vehicles.
With support for 27 languages, PicoScope 7 software allows easy global collaboration. PicoScope 7 is free to download on Pico’s website.
Find more datasheets on products like this one at Datasheets.com, searchable by category, part #, description, manufacturer, and more.
The post PC-based scopes gain 10Base-T1S decoder appeared first on EDN.
Sensor enables ghost-free HDR imaging

The OG0TC global-shutter image sensor from Omnivision brings the company’s DCG high dynamic range technology to AR/VR/MR tracking cameras. Intended for eye and face tracking, the backside-illuminated sensor’s on-chip single-exposure DCG extends dynamic range up to 140 dB, ensuring images are free of ghosting and motion artifacts.
Based on a stacked-die construction, the OG0TC sensor is just 1.64×1.64 mm. It offers a resolution of 400×400 pixels with a pixel size of 2.2 µm in a 1/14.46-in. optical format. This small, low-power CMOS sensor is designed primarily for inward-facing tracking cameras. Its small form factor is key to AR/VR designs, as multiple cameras are required for tracking all aspects of the face (eyes, brows, lips).
Ultra-low power consumption is crucial for AR/VR devices. The OG0TC image sensor cuts power usage by over 40% compared to the previous-generation OG0TB, while maintaining pin-to-pin compatibility for easy upgrades and adding features like DCG technology, according to Devang Patel, Marketing Director of IoT/Emerging, Omnivision.
Offered in a 16-pin chip-scale package, the OG0TC global-shutter image sensor is now available for sampling and in mass production.
Find more datasheets on products like this one at Datasheets.com, searchable by category, part #, description, manufacturer, and more.
The post Sensor enables ghost-free HDR imaging appeared first on EDN.
64-bit MPUs advance space computing

Microchip has launched the first devices in its PIC64 High-Performance Spaceflight Computing (PIC64-HPSC) family of microprocessors. These multicore 64-bit RISC-V processors, which Microchip is delivering to NASA and the broader defense and commercial aerospace industry, employ vector-processing instruction extensions to support AI and ML. They also offer features and industry-standard interfaces not previously available for space applications.
Radiation-hardened PIC64-HPSC RH MPUs provide autonomous missions with the local processing power needed to execute real-time tasks. They can be used for rover hazard avoidance on the moon’s surface, as well as long-duration deep-space missions like Mars expeditions.
Radiation-tolerant PIC64-HPSC RT MPUs are tailored for the commercial space sector, particularly Low Earth Orbit (LEO) constellations. They balance cost-effectiveness with high fault tolerance crucial for round-the-clock service reliability and space asset cybersecurity.
The space-grade architecture of these processors includes eight SiFive RISC-V X280 64-bit CPU cores. They support virtualization and real-time operation, with vector extensions capable of delivering up to 2 TOPS (Int8) or 1 TFLOPS (Bfloat16) for autonomous missions.
PIC64-HPSC devices also provide high-speed network connectivity, low-latency data transfers, and platform-level defense-grade security. Dynamic controls manage computational demands across different phases of space missions, activating functions and interfaces as needed.
Samples of the PIC64-HPSC processors will be available to early access partners in 2025. For additional information, contact a Microchip sales representative.
Find more datasheets on products like this one at Datasheets.com, searchable by category, part #, description, manufacturer, and more.
The post 64-bit MPUs advance space computing appeared first on EDN.
Makers and Learners Rejoice! Arduino Debuts Plug and Make Kit
Automate battery management system (BMS) test with a digital twin

A battery management system (BMS) monitors and controls batteries in vehicles such as more-electric aircraft and electric cars. It needs to undergo rigorous tests under nominal and extreme conditions to prove its quality and integrity.
Testing with emulated battery cells is beneficial because one can safely test all kinds of conditions quickly and repeatedly without risking precious hardware. This type of hardware-in-the-loop testing simplifies quality assurance and keeps up with the pace of innovation.
Batteries are crucial for electrifying drive trains in vehicles or actuators in aircraft and ships, and BMS is a vital piece of the puzzle for controlling and monitoring the battery pack. The BMS ensures safe battery operation, effective use of its capacity, and long service life.
BMS is used in cars, aircraft, energy storage systems, and consumer electronics, among other things. It typically comprises a battery management unit (BMU), a cell monitoring unit (CMU), and a power distribution unit (PDU).
Figure 1 BMS ensures safe battery operation, effective use of its capacity, and long service life. Source: Speedgoat GmbH
The battery management unit is the main controller; being connected to the cell monitoring and power distribution unit, it monitors the overall state of charge (SOC) as well as cell voltages and cell temperature information. The cell monitoring unit is linked to the battery cells; these modules form battery packs—each has a CMU to regulate the charge and discharge of individual cells, temperature, and voltage.
The power distribution unit is connected to all components that draw power from the pack or feed it back in—in an electric vehicle (EV), this could be the charging system and the motor, for example.
Testing battery management system (BMS)
Rigorous testing ensures that BMS fulfills its requirements, such as optimally distributing the current between the cells during charging. The BMS is checked in the nominal range and extreme situations, for example, when cells overheat, a signal fails, or a short circuit occurs. This way, it’s possible to test how BMS reacts in such cases and ensure correct functioning.
Testing BMS comes with various challenges and is a complex task. The BMS houses various controllers, processes signals from distributed sensors and is linked to numerous systems such as the powertrain. Testing all functionalities, configurations, and states is a lot of effort, which is scarcely achievable with real batteries.
As batteries age, it’s also vital to reproduce conditions repeatedly to test critical algorithms such as cell balancing and state of charge or state of health (SoH) estimation. In addition, different teams are usually responsible for the individual components during development, and their engineers are not always available.
Batteries, BMS and infrastructures are also evolving, so the providers of the systems must keep up with this and react swiftly to new requirements. Finally, testing with real hardware can be dangerous; in the worst case, batteries could explode due to over-voltages or extreme temperatures.
BMS testing with digital twins
These challenges can be solved using a battery’s digital twin. A battery cell emulator can mimic the behavior of the battery by precisely emulating voltages, current levels, and temperatures. It can represent various battery pack architectures and integrate seamlessly with standard test frameworks.
Figure 2 The Battery Cell Emulator (BCE) mimics the behavior of the battery by precisely emulating voltages, current levels, and temperatures. Source: Speedgoat GmbH
Taking into account battery technology and chemistry, age, and operating temperatures, the battery cell emulator can accommodate all kinds of battery models. Tests can be conducted swiftly and safely in regular operational conditions and under faulty conditions.
The same communication protocols are used when interfacing with the actual batteries. Testing with a digital twin also facilitates testing the rest of the system, such as power distribution and charging components, motor drives and fuel cells.
It must be possible to reproduce the same conditions in tests to check the controllers’ behavior reliably. In addition, a flexible test infrastructure that allows engineers to continuously test functionalities and changes in the development process to fulfill novel requirements is essential. Furthermore, testing many scenarios, including complexities, is crucial to achieve complete test coverage.
Test cases are usually defined and tracked in software. Automated test procedures are important for repeating tests and comparing results efficiently. Requirements are typically managed in Simulink’s dedicated toolboxes, such as Simulink Test, or ASAM XIL-compatible third-party software tools.
The advantages of digital testing
For testing the BMS controller and its interactions with various components, the behavior of the actual battery can be precisely emulated with a digital twin. Many insights are available early, allowing the engineers to adjust designs and functions when changes are still easy to implement. Also, a battery’s digital twin does justice to fast-changing trends—it’s more flexible than hardware. It can be configured seamlessly to adapt to new conditions.
Additionally, with the digital twin, components can be tested before assembly. This saves time, and complications or design errors can be found early in the development process, improving the quality and speed of development. Likewise, development and testing costs can be saved because corrections are made at early stages rather than late in development. Finally, risk-free testing of the BMS is possible in extreme events such as collisions, faulty cells, or over-voltage.
Therefore, testing with digital twins of batteries is suitable for engineers who test and validate battery management systems and aim for a continuous and automated workflow. An attractive feature for users is that they can carry out all tests in one software environment (Simulink).
The battery model is developed in Simulink. Afterward, the tests can be performed using the same model with the hardware in the background. The flexible test infrastructure and toolboxes enable continuous, automated testing along the defined requirements.
Figure 3 All automated BMS tests can be performed in a single software environment (Simulink). Source: Speedgoat GmbH
As battery management systems ensure safe battery operation, they need to be thoroughly tested. A digital twin allows engineers to precisely emulate BMS batteries and to safely test all kinds of conditions quickly and repeatedly without risking damaging hardware. Such a test system is suitable for engineers in various industries who need to conduct BMS tests continuously and automatically,
Nadja Müller is a freelance journalist specializing in digitalization.
Related Content
- How to design a battery management system
- BMS Innovations Will Require New EV Test Solutions
- The ins and outs of battery management system (BMS)
- Perform functional testing of battery management systems for hybrid electric vehicles
- High-Performance Electromobility Solutions with Battery Management and Embedded Technology
The post Automate battery management system (BMS) test with a digital twin appeared first on EDN.
Transforming Devices into Smart Innovations: NeoMesh Sensor Modules Power Endrich Bauelemente’s Smart Fridge Concept
According to a recent Statista report, global spending on Internet of Things (IoT) products reached $805 billion in 2023, with expectations for continued growth in the coming years. For several years, Endrich Bauelemente GmbH, the German distributor for NeoCortec, has been engaged in developing smart IoT-related products, such as a concept for a smart fridge. They have incorporated NeoCortec’s ultra-low-power, scalable NeoMesh wireless sensor modules into their solutions.
One major advantage of a mesh solution like NeoMesh technology is that it requires only one central gateway to collect and transmit all sensor data to the Cloud. Connecting different parts of the network does not require repeaters or additional gateways. Zoltan Kiss, Head of the R&D Department at Endrich Bauelemente, explains, “By integrating NeoMesh sensor modules into our solution, we can easily capture necessary data and wirelessly transmit it to our own IoT ecosystem or any other suitable cloud service.” Kiss adds, “With its extremely low power consumption and ability to establish scalable local wireless networks, NeoMesh is the ideal product for our smart IoT solution.”
In partnership with NeoCortec, Endrich Bauelemente GmbH has been actively developing a smart fridge concept to enable manufacturers to incorporate intelligent features, allowing end users to monitor various parameters of their refrigerators via a mobile application.
The NeoMesh wireless sensor modules facilitate seamless and efficient integration of multiple IoT functionalities. These functionalities include monitoring temperature and humidity inside the refrigerator, as well as tracking interior light brightness and door status. Data collection on the frequency and duration of door openings can provide valuable insights for marketing or commercial purposes, especially in settings like gas stations or retail stores. One of Endrich’s initial clients, Audax Electronics in Brazil, has successfully integrated NeoMesh modules into the LED lighting units of their fridges to monitor temperature and door status. These LED units can be installed in both smart-capable and conventional refrigerators.
Key requirements for IoT sensor modules in smart devices include compact size, easy installation, independence from electrical and wired communication networks, and straightforward commissioning. Battery-powered, wireless communication technology like NeoMesh, with all necessary sensors integrated into the module, allows for installation without a specialist. “This user-friendliness is what makes our NeoMesh technology so appealing,” comments Thomas Steen Halkier, CEO of NeoCortec. Sensor measurements are wirelessly transmitted to the appropriate cloud service for data analysis via the self-forming mesh network. This eliminates the need for extensive cabling, reducing infrastructure costs and providing greater flexibility in network deployment, as sensor nodes can be installed anywhere. “Our NeoMesh technology is especially suited for wireless sensor networks where sensors don’t need to transmit data frequently and where the data payload size is small,” adds Halkier.
The NeoMesh wireless communication protocol is supported by a variety of fully-integrated, pre-certified ultra-low-power bi-directional sensor modules. These modules come in various versions, all incorporating the core NeoMesh protocol stack across different frequency bands (868 MHz, 915 MHz, and 2.4 GHz) and preloaded with the proprietary NeoCortec protocol stack.
The post Transforming Devices into Smart Innovations: NeoMesh Sensor Modules Power Endrich Bauelemente’s Smart Fridge Concept appeared first on ELE Times.
What’s next in on-device generative AI?
Upcoming generative AI trends and Qualcomm Technologies’ role in enabling the next wave of innovation on-device
The generative artificial intelligence (AI) era has begun. Generative AI innovations continue at a rapid pace and are being woven into our daily lives to offer enhanced experiences, improved productivity and new forms of entertainment. So, what comes next? This blog post explores upcoming trends in generative AI, advancements that are enabling generative AI at the edge and a path to humanoid robots. We’ll also illustrate how Qualcomm Technologies’ end-to-end system philosophy is at the forefront of enabling this next wave of innovation on-device.
Upcoming trends and why on-device AI is key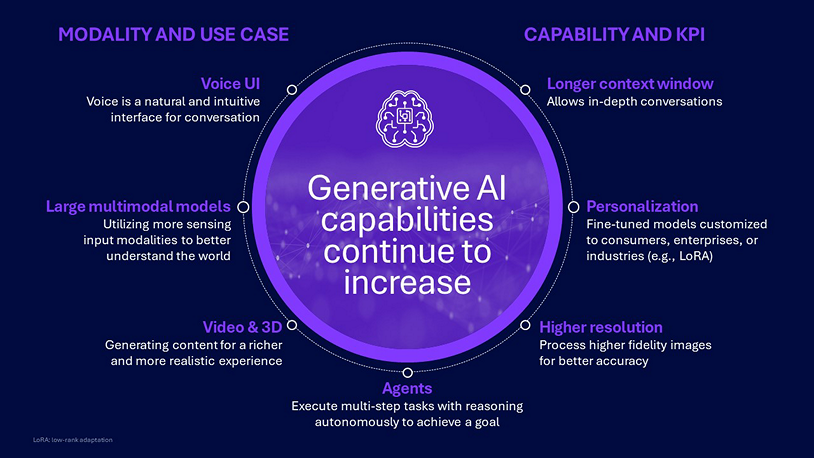
dimensions.
Transformers, with their ability to scale, have become the de facto architecture for generative AI. An ongoing trend is transformers extending to more modalities, moving beyond text and language to enable new capabilities. We’re seeing this trend in several areas, such as in automotive for multi-camera and light detection and ranging (LiDAR) alignment for bird’s-eye-view or in wireless communications where global position system (GPS), camera and millimeter wave (mmWave) radio frequency (RF) are combined using transformers to improve mmWave beam management.
Another major trend is generative AI capabilities continuing to increase in two broad categories:
- Modality and use case
- Capability and key performance indicators (KPIs)
For modality and use cases, we see improvements in voice user interface (UI), large multimodal models (LMMs), agents and video/3D. For capabilities and KPIs, we see improvements for longer context window, personalization and higher resolution.
In order for generative AI to reach its full potential, bringing the capabilities of these trends to edge devices is essential for improved latency, pervasive interaction and enhanced privacy. As an example, enabling humanoid robots to interact with their environment and humans in real time requires on-device processing for immediacy and scalability.
Advancements in edge platforms for generative AIHow can we bring more generative AI capabilities to edge devices?
We are taking a holistic approach to advance edge platforms for generative AI through research across multiple vectors.
We aim to optimize generative AI models and efficiently run them on hardware through techniques such as distillation, quantization, speculative decoding, efficient image/video architectures and heterogeneous computing. These techniques can be complementary, which is why it is important to attack the model optimization and efficiency challenge from multiple angles.
Consider quantization for large language models (LLMs). LLMs are generally trained in floating-point 16 (FP16). We’d like to shrink an LLM for increased performance while maintaining accuracy. For example, reducing the FP16 model to 4-bit integer (INT4), reduces the model size by four times. That also reduces memory bandwidth, storage, latency and power consumption.
Quantization-aware training with knowledge distillation helps to achieve accurate 4-bit LLMs, but what if we need an even lower number of bits per value? Vector quantization (VQ) can help with this. VQ shrinks models while maintaining desired accuracy. Our VQ method achieves 3.125 bits per value at similar accuracy as INT4 uniform quantization, enabling even bigger models to fit within the dynamic random-access memory (DRAM) constraints of edge devices.
Another example is efficient video architecture. We are developing techniques to make generative video methods efficient for on-device AI. As an example, we optimized FAIRY, a video-to-video generative AI technique. In the first stage of FAIRY, states are extracted from anchor frames. In the second stage, video is edited across the remaining frames. Example optimizations include: cross-frame optimization, efficient instructPix2Pix and image/text guidance conditioning.
A path to humanoid robotsWe have expanded our generative AI efforts to study LLMs and their associated use cases, and in particular the incorporation of vision and reasoning for large multimodal models (LMMs). Last year, we demonstrated a fitness coach demo at CVPR 2023, and recently investigated the ability of LMMs to reason across more complex visual problems. In the process, we achieved state-of-the-art results to infer object positions in the presence of motion and occlusion.
However, open-ended, asynchronous interaction with situated agents is an open challenge. Most solutions for LLMs right now have basic capabilities:
- Limited to turn-based interactions about offline documents or images.
- Limited to capturing momentary snapshots of reality in a Visual Question Answering-style (VQA) dialogue.
We’ve made progress with situated LMMs, where the model is able to process a live video stream in real time and dynamically interact with users. One key innovation was the end-to-end training for situated visual understanding — this will enable a path to humanoids.
More on-device generative AI technology advancements to comeOur end-to-end system philosophy is at the forefront of enabling this next wave of innovation for generative AI at edge. We continue to research and quickly bring new techniques and optimizations to commercial products. We look forward to seeing how AI ecosystem leverages these new capabilities to make AI ubiquitous and to provide enhanced experiences.
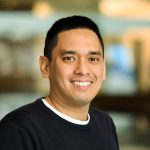
Senior Director of Technology,
Qualcomm Technologies
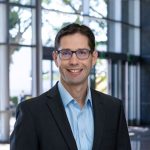
The post What’s next in on-device generative AI? appeared first on ELE Times.
Advanced Material Handling: The Flexible Transport System (FTS) from Rexroth
The Flexible Transport System (FTS) from Bosch Rexroth is an innovative solution designed for the precise transport and positioning of materials and workpieces. It is a magnetically propelled transport platform designed to enhance pallet speed and positioning accuracy, especially for heavier loads like battery modules.
Traditional rollers, chains, or belt systems often fall short in demanding applications, but the FTS overcomes these limitations with exceptional accuracy, programmable movements, and superior speed. The non-contact drive concept ensures particle-free transport, even in vacuum environments.
FTS Features at a Glance- Extremely Precise
The FTS achieves remarkable positioning accuracy and high repeatability, thanks to its advanced sensors placed between individual motors and a sophisticated motion control system. This precision is critical in applications requiring meticulous material handling.
- Individually Scalable
The system’s scalability allows it to meet various production size requirements. It can be easily expanded with multiple motors to accommodate longer production lines. The carriers are designed to handle both heavy and light objects with equal precision, making the FTS a versatile solution for diverse industries.
- Flexibly Adaptable
Offering maximum flexibility, the FTS allows for the free programmability of all carrier movements, including I/O synchronization if needed. This adaptability facilitates quick conversions to different products, ensuring seamless transitions and reducing downtime. The mechanical components of the system are designed to integrate effortlessly with various machines.
System DescriptionThe FTS provides the flexibility to build a system tailored to specific needs, whether as a standalone unit or integrated into an existing production line. The open system design enables carriers to transition smoothly between external conveyor belts and the FTS. Additionally, robots can be strategically placed along the tracks to perform assembly tasks in conjunction with the FTS. It features interfaces in C/C++ or PLC with standard Ethernet, and since the software operates on a standard PC, other interfaces can also be incorporated.
HardwareThe FTS’s hardware is based on the embedded control YM, offering unparalleled design freedom. This next-generation hardware is engineered to handle complex operations, and its open software architecture supports the creation of customized motion solutions that integrate seamlessly into existing automation landscapes. The compact modular multi-axis controller contains all necessary control and drive hardware, facilitating precise control and high-speed operations.
High-level programming languages enable the development of intricate motion control programs, while high-speed control loops with 32 kHz bandwidth ensure pinpoint accuracy and performance. This hardware configuration supports complex, high-precision tasks with ease.
SoftwareThe core of the FTS’s powerful and flexible technology is its intelligent motion control system from Rexroth. This system combines high-performance hardware capable of managing complex processes with open software structures that allow for customized movements. The software integrates effortlessly into existing automation systems, providing a robust and adaptable solution for various applications.
The control platform includes advanced diagnostics, error analysis, and maintenance capabilities. It continuously monitors carrier positions, current, position errors, and motion profiles, providing real-time data visualization through its toolset. This comprehensive monitoring ensures optimal performance and facilitates prompt issue resolution, maintaining smooth and efficient operations.
Rexroth’s FTS stands out as a versatile, precise, and adaptable solution for advanced material transport and positioning, meeting the needs of demanding applications with unparalleled efficiency and reliability.
The post Advanced Material Handling: The Flexible Transport System (FTS) from Rexroth appeared first on ELE Times.
AI-Powered Battery System on Chip: A Masterstroke in Battery Management System
Eatron Technologies has introduced its latest breakthrough in battery management technology—a state-of-the-art AI-powered Battery Management System on Chip, developed in partnership with Syntiant. This ground-breaking solution merges Eatron’s sophisticated Intelligent Software Layer with Syntiant’s ultra-low power NDP120 Neural Decision Processor, delivering unmatched battery performance, safety, and longevity.
The AI-BMS-on-chip marks a major advancement in battery management. This powerful yet energy-efficient system unlocks an additional 10% of battery capacity and extends battery life by up to 25%. By integrating their pre-trained AI models, the solution offers state-of-health, state-of-charge, and remaining useful life assessments with remarkable accuracy right out of the box.
Key Benefits- Enhanced Performance: This solution optimizes available battery power by providing precise state-of-charge and health estimations.
- Improved Safety: Early detection of potential issues through predictive diagnostics ensures operational safety and prevents failures.
- Increased Longevity: By effectively managing battery health and usage, this solution extends the lifespan of batteries.
A standout feature of the AI-BMS-on-chip is its capability to perform real-time analysis and decision-making directly on the device. By harnessing the efficient processing capabilities of Syntiant’s NDP120, this solution operates at the edge, thereby obviating the requirement for intricate cloud infrastructure. This results in reduced latency, lower power consumption, and overall system costs.
Versatile and Easy to IntegrateDesigned for seamless integration, the AI-BMS-on-chip enhances performance, safety, and longevity across a wide range of battery-powered applications, including light mobility, industrial, and consumer electronics. In addition to expediting time-to-market, this plug-and-play solution offers customization capabilities through an intuitive toolchain, tailoring it precisely to individual applications. Existing BMS hardware can be easily upgraded to benefit from this best-in-class performance, providing a cost-effective solution for businesses striving to stay competitive.
Pioneering Collaboration: Transforming Battery ManagementEatron Technologies and Syntiant have been collaborating since 2022, merging their expertise in battery management and AI technologies. Amedeo Bianchimano, Chief Product Delivery Officer at Eatron Technologies, highlighted, “The AI-BMS-on-chip empowers the safe and efficient deployment of any battery-powered application, optimizing battery energy usage.” In agreement, Mallik P. Moturi, Chief Business Officer at Syntiant Corp., emphasized, “Through our NDP120, Eatron’s software processes all data at the edge, boosting battery life, safety, and overall performance. This makes it ideal for everything from consumer electronics to commercial vehicles.”
This collaboration represents a new chapter in battery management, providing unprecedented performance, safety, and longevity to a variety of applications. Eatron Technologies and Syntiant are proud to lead the way in this innovative field, offering cutting-edge solutions that address the evolving needs of the industry.
The post AI-Powered Battery System on Chip: A Masterstroke in Battery Management System appeared first on ELE Times.
Key Design Considerations for Offline SMPS Applications
Courtesy: Onsemi
Every electronic device that is powered from a wall outlet uses some form of offline switch mode power supply (SMPS) that converts the AC grid voltage to a DC voltage used by the device. An offline SMPS is a switched power supply with an isolation transformer and covers power range from a few watts to multi-kilowatt solutions. Offline SMPS is widely deployed and indispensable in providing reliable and safe power to electronic devices in various applications ranging from consumer electronics, industrial power supply, datacenters to telecom base stations.
When designing an offline SMPS there are many factors to be considered for a successful design including power level, voltages, safety requirements, size, and several more.
Understanding Offline SMPS & Popular TopologiesFundamentally, an online SMPS uses a two-stage conversion. Firstly, the mains grid voltage is rectified and shaped by the first stage – the power factor corrector (PFC). The output voltage of the PFC stage is set to be a bit higher than the expected input peak voltage. For single phase solutions this is usually around 380-400 VDC. Since the output of the PFC stage is stable and relatively well-regulated DC voltage, the following DC-DC stage can be less complex. In most offline SMPS, the PFC is single-phase, but for higher power units (multi-kilowatt) it can be 3-phase.
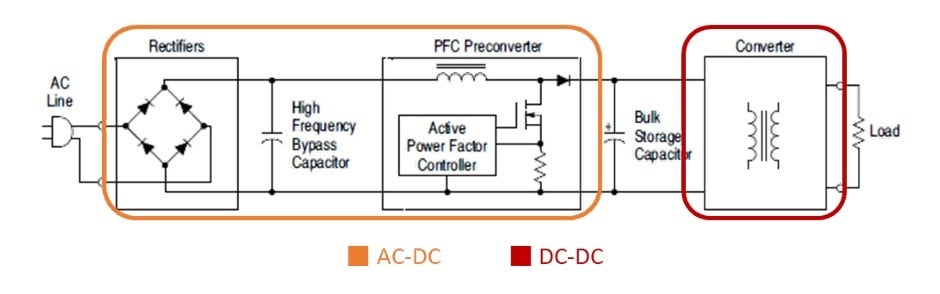
The PFC stage aims to improve efficiency by reducing the apparent power in the system. It corrects the phase difference between the current and voltage (the ‘Power Factor’) to maintain as little difference as possible, as well as shaping the current waveform to be as near as it can be to a pure sinusoid, minimizing total harmonic distortion (THD).
The DC-DC stage (often an LLC converter) takes the PFC output and converts this to the desired voltage, bearing in mind there may be several independent outputs. This stage also includes the galvanic isolation transformer that provides safety isolation as well as level shifting the voltage. Due to the transformer’s inability to accommodate direct current, the incoming DC from the PFC stage is converted back into an alternating current and then rectified for the output.
Efficiency (the ratio between the power delivered at the output and the power consumed by the input) is a crucial parameter for any SMPS. It affects the operating cost, but more importantly, it also defines the internal losses that manifest as heat. This, in turn, determines how much cooling is required when the SMPS is operating. The higher the amount of cooling in terms of fans and/or heatsinks is needed, the larger, heavier and more expensive the solution will be.
Advancements in Offline SMPS TechnologyStriving for the highest levels of performance, there is ongoing advancement in the technologies used within offline SMPS.
Boost PFC is nowadays commonly used for a wide range of power due to its simple structure and straightforward control strategy. The inductor current is continuous, electromagnetic interference (EMI) is lower, and the current waveform is less distorted, which leads to a better power factor. A single-phase boost PFC will have a regulated DC output of around 380 V, which will then be converted by the DC/DC converter.
Furthermore, LLC converters are becoming increasingly popular for the DC-DC stage. These resonant converters regulate their output by altering the operating frequency of the resonant tank across a relatively narrow range, thereby operating in a soft-switching mode. This improves efficiency and reduces EMI. They operate at higher frequencies compared to allowing the use of smaller passive components.
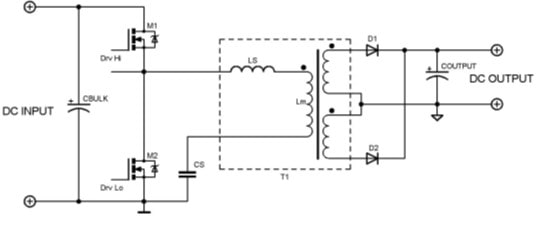
Synchronous or active rectification is a technique for improving efficiency and reducing conduction losses by replacing rectifier diodes with active switches. While semiconductor diodes exhibit a relatively fixed voltage drop (typically 0.5 to 1 V), MOSFET switches act as resistances and therefore can have very low drop. If further improvements are needed, MOSFET switches can be paralleled in order to handle higher output currents. In such a case the conduction losses are reduced, because the RDSON of the paralleled devices is equal to the inverse sum of their respective RDSON.
Semiconductor materials are also evolving as traditional silicon (Si) has reached its limit for further significant performance gains. New wide-bandgap (WBG) materials such as silicon carbide (SiC) are increasingly preferred in power designs for their ability to operate efficiently at higher switching frequencies and higher operating voltages.
WBG devices exhibit lower losses due to better reverse recovery, significantly contributing to enhanced conversion efficiency. As a result, and due to their ability to operate at higher temperatures, thermal mitigation requirements are reduced when using WBG devices.
onsemi Solutionsonsemi has one of the broadest portfolios of solutions for offline SMPS currently available. At the heart of the range are controllers for the PFC and DC/DC converter stages, power MOSFETs, rectifiers, and diodes. This is supported with MOSFET gate drivers (including for synchronous rectification), optocouplers, low dropout (LDO) regulators, and other devices.
Leading the way in modern high-performance devices, the range includes many SiC devices (diodes and MOSFETs) for use in the most challenging offline SMPS applications.
Using the onsemi range (and a few passive components), offline SMPS from a few watts to several kilowatts can be designed. onsemi’s experience in this area assures designers that their solution will have industry-leading performance and reliability.
ConclusionOffline SMPS are one of the most common sub-systems, present in almost every mains-connected device. However, to create a successful design, safety, and EMI regulations must be met while performance, especially in terms of efficiency, is an ever-increasing requirement.
While several companies manufacture some of the devices necessary for these designs, few (if any) have a comprehensive range that covers all the components (excluding passives) needed to execute a complete design. There are significant benefits to sourcing components from a single supplier, including knowing that devices have been designed and tested to work together.
The post Key Design Considerations for Offline SMPS Applications appeared first on ELE Times.
TOPS of the Class: Decoding AI Performance on RTX AI PCs and Workstations
Courtesy: Nvidia
What is a token? Why is batch size important? And how do they help determine how fast AI computes?The era of the AI PC is here, and it’s powered by NVIDIA RTX and GeForce RTX technologies. With it comes a new way to evaluate performance for AI-accelerated tasks, and a new language that can be daunting to decipher when choosing between the desktops and laptops available.
While PC gamers understand frames per second (FPS) and similar stats, measuring AI performance requires new metrics.
Coming Out on TOPSThe first baseline is TOPS, or trillions of operations per second. Trillions is the important word here — the processing numbers behind generative AI tasks are absolutely massive. Think of TOPS as a raw performance metric, similar to an engine’s horsepower rating. More is better.
Compare, for example, the recently announced Copilot+ PC lineup by Microsoft, which includes neural processing units (NPUs) able to perform upwards of 40 TOPS. Performing 40 TOPS is sufficient for some light AI-assisted tasks, like asking a local chatbot where yesterday’s notes are.
But many generative AI tasks are more demanding. NVIDIA RTX and GeForce RTX GPUs deliver unprecedented performance across all generative tasks — the GeForce RTX 4090 GPU offers more than 1,300 TOPS. This is the kind of horsepower needed to handle AI-assisted digital content creation, AI super resolution in PC gaming, generating images from text or video, querying local large language models (LLMs) and more.
Insert Tokens to PlayTOPS is only the beginning of the story. LLM performance is measured in the number of tokens generated by the model.
Tokens are the output of the LLM. A token can be a word in a sentence, or even a smaller fragment like punctuation or whitespace. Performance for AI-accelerated tasks can be measured in “tokens per second.”
Another important factor is batch size, or the number of inputs processed simultaneously in a single inference pass. As an LLM will sit at the core of many modern AI systems, the ability to handle multiple inputs (e.g. from a single application or across multiple applications) will be a key differentiator. While larger batch sizes improve performance for concurrent inputs, they also require more memory, especially when combined with larger models.
The more you batch, the more (time) you save.
RTX GPUs are exceptionally well-suited for LLMs due to their large amounts of dedicated video random access memory (VRAM), Tensor Cores and TensorRT-LLM software.
GeForce RTX GPUs offer up to 24GB of high-speed VRAM, and NVIDIA RTX GPUs up to 48GB, which can handle larger models and enable higher batch sizes. RTX GPUs also take advantage of Tensor Cores — dedicated AI accelerators that dramatically speed up the computationally intensive operations required for deep learning and generative AI models. That maximum performance is easily accessed when an application uses the NVIDIA TensorRT software development kit (SDK), which unlocks the highest-performance generative AI on the more than 100 million Windows PCs and workstations powered by RTX GPUs.
The combination of memory, dedicated AI accelerators and optimized software gives RTX GPUs massive throughput gains, especially as batch sizes increase.
Text-to-Image, Faster Than EverMeasuring image generation speed is another way to evaluate performance. One of the most straightforward ways uses Stable Diffusion, a popular image-based AI model that allows users to easily convert text descriptions into complex visual representations.
With Stable Diffusion, users can quickly create and refine images from text prompts to achieve their desired output. When using an RTX GPU, these results can be generated faster than processing the AI model on a CPU or NPU.
That performance is even higher when using the TensorRT extension for the popular Automatic1111 interface. RTX users can generate images from prompts up to 2x faster with the SDXL Base checkpoint — significantly streamlining Stable Diffusion workflows.
ComfyUI, another popular Stable Diffusion user interface, added TensorRT acceleration last week. RTX users can now generate images from prompts up to 60% faster, and can even convert these images to videos using Stable Video Diffuson up to 70% faster with TensorRT.
TensorRT acceleration can be put to the test in the new UL Procyon AI Image Generation benchmark, which delivers speedups of 50% on a GeForce RTX 4080 SUPER GPU compared with the fastest non-TensorRT implementation.
TensorRT acceleration will soon be released for Stable Diffusion 3 — Stability AI’s new, highly anticipated text-to-image model — boosting performance by 50%. Plus, the new TensorRT-Model Optimizer enables accelerating performance even further. This results in a 70% speedup compared with the non-TensorRT implementation, along with a 50% reduction in memory consumption.
Of course, seeing is believing — the true test is in the real-world use case of iterating on an original prompt. Users can refine image generation by tweaking prompts significantly faster on RTX GPUs, taking seconds per iteration compared with minutes on a Macbook Pro M3 Max. Plus, users get both speed and security with everything remaining private when running locally on an RTX-powered PC or workstation.
The Results Are in and Open SourcedBut don’t just take our word for it. The team of AI researchers and engineers behind the open-source Jan.ai recently integrated TensorRT-LLM into its local chatbot app, then tested these optimizations for themselves.
The researchers tested its implementation of TensorRT-LLM against the open-source llama.cpp inference engine across a variety of GPUs and CPUs used by the community. They found that TensorRT is “30-70% faster than llama.cpp on the same hardware,” as well as more efficient on consecutive processing runs. The team also included its methodology, inviting others to measure generative AI performance for themselves.
From games to generative AI, speed wins. TOPS, images per second, tokens per second and batch size are all considerations when determining performance champs.
The post TOPS of the Class: Decoding AI Performance on RTX AI PCs and Workstations appeared first on ELE Times.
Canada invests $120m to support semiconductor manufacturing and commercialization
Luminus launches high-efficacy mid-power MP-5050 LEDs
Accenture Acquires Cientra to Expand Silicon Design Capabilities
Accenture has acquired Cientra, a silicon design and engineering services company, offering custom silicon solutions for global clients. The terms of the acquisition were not disclosed.
Founded in 2015, Cientra is headquartered in New Jersey, U.S. and has offices in Frankfurt, Germany as well as in Bangalore, Hyderabad and New Delhi, India. The company brings consulting expertise in embedded IoT and application-specific integrated circuit design and verification capabilities, which augments Accenture’s silicon design experience and further enhances its ability to help clients accelerate semiconductor innovation required to support growing data computing needs.
“Everything from data center expansion to cloud computing, wireless technologies, edge computing and the proliferation of AI, are driving demand for next-generation silicon products,” said Karthik Narain, group chief executive—Technology at Accenture. “Our acquisition of Cientra is our latest move to expand our silicon design and engineering capabilities and it underscores our commitment to helping our clients maximize value and reinvent themselves in this space.”
Cientra has deep experience in engineering, development and testing across hardware, software and networks, in the automotive, telecommunications and high-tech industries. The company brings approximately 530 experienced engineers and practitioners to Accenture’s Advanced Technology Centers in India.
“Since inception, Cientra has been dedicated to building top talent and fostering continuous innovation, developing product solutions that drive value for our clients,” said Anil Kempanna, CEO, Cientra. “Joining Accenture provides exciting opportunities to expand globally and scale our capabilities to create new avenues of growth for our clients as well as our people.”
This acquisition follows the addition of Excelmax Technologies, a Bangalore, India-based semiconductor design services provider, earlier this week, and XtremeEDA, an Ottawa, Canada-based silicon design services company, in 2022.
The post Accenture Acquires Cientra to Expand Silicon Design Capabilities appeared first on ELE Times.
EPC Space showcasing rad-hard solutions at NSREC 2024
E-Fill Electric Presents Wish List for EV Charging Industry in the Upcoming Union Budget
E-Fill Electric (EFEV Charging Solutions Pvt. Ltd.), a pioneering name in India’s EV charging sector, has outlined its wish list for the forthcoming Union Budget, emphasizing key actions to promote the adoption and infrastructure development of electric vehicles (EVs) across the country.
Mayank Jain, Founder & CEO of E-Fill Electric, highlighted several key priorities aimed at fostering a robust EV charging ecosystem:
- Increased Allocation for FAME Scheme: E-Fill Electric urges the government to enhance allocation under the FAME (Faster Adoption and Manufacturing of Electric Vehicles) scheme to accelerate EV adoption and manufacturing capabilities in India.
- Tax Incentives for EV Charging Businesses: Lowering GST on EV charging equipment and operational costs shall ensure affordability and promote widespread deployment of charging infrastructure.
- Investment in Skilled Workforce: E-Fill Electric stresses the importance of investing in training programs to develop a skilled workforce proficient in EV charging installation, maintenance, and repair, vital for sustaining the industry’s growth.
- Streamlined Land Acquisition Procedures: The Company recommends measures to streamline land acquisition procedures for EV charging companies, potentially through designated zones or expedited approvals, to facilitate speedy infrastructure expansion.
- Public-Private Partnerships: E-Fill Electric advocates for incentivising partnerships between public and private entities to expedite the development and deployment of EV charging infrastructure nationwide.
- Research and Development Incentives: The budget should incentivise research and development in EV charging technology, including support for indigenous manufacturing of charging equipment to encourage innovation and self-reliance.
- Subsidies for EV Chargers: E-Fill Electric suggests introducing subsidies or low-interest loan schemes to encourage individuals and businesses to install EV chargers at homes and workplaces, enhancing convenience and accessibility.
- Grid Modernization: Prioritizing grid modernisation projects is essential to accommodate the increased electricity demand from EVs, ensuring reliable and sustainable power supply.
Mr. Mayank Jain expressed confidence that these measures, if implemented, will not only boost the EV ecosystem but also align with India’s vision of sustainable and inclusive mobility solutions.
The post E-Fill Electric Presents Wish List for EV Charging Industry in the Upcoming Union Budget appeared first on ELE Times.
ROHM Offers LogiCoA: the Industry’s First* Analog-Digital Fusion Control Power Supply Solution
Provides functions equivalent to a fully digital control power supply with low power consumption
ROHM has established LogiCoA, a power supply solution for small to medium-power industrial and consumer equipment (30W to 1kW class). It provides the same functionality as fully digital control power supplies at low power consumption and cost equivalent to analog power types.
Analog controlled power supplies are commonly used in industrial robotics and semiconductor manufacturing equipment that operate in the medium power range. However, in recent years these power supplies are also required to provide a high level of reliability and precise control that make it difficult to meet market demands with analog-only configurations. On the other hand, while fully digitally controlled power supplies enable fine control and settings, they are not widely adopted in the small to medium power range due to the high power consumption and cost of the digital controller. To address this issue, ROHM developed the LogiCoA power solution that leverages the strengths of both analog and digital technologies. High-performance low power LogiCoA MCUs are utilized to facilitate control of a variety of power supply topologies.
The LogiCoA brand embodies a design philosophy of fusing digital elements to maximize the performance of analog circuits. ROHM’s LogiCoA power solution is the industry’s first* “analog-digital fusion control” power supply that combines a digital control block centered around the LogiCoA MCU with analog circuitry comprised of silicon MOSFETs and other power devices.
In a fully digital control power supply, the functions handled by digital controllers such as high-speed CPUs or DSPs can be processed by low-bit MCUs, making it possible to achieve increased functionality that is difficult to realize with an analog control power supply at low power consumption and cost. This solution allows for the correction of performance variations in peripheral components according to the power supply circuit by storing various settings such as current and voltage values in the LogiCoA MCU. As a result, there is no need to consider design margins unlike with analog control power supplies, contributing to smaller power supplies that provide greater reliability. On top, as operation log data can be recorded in the MCU’s nonvolatile memory, it is ideal for power supplies in industrial equipment that require logging as a backup in case of malfunction.
The evaluation reference design REF66009 allows users to experience the LogiCoA power supply solution in a non-isolated buck converter circuit. Various tools necessary for evaluation are also offered, including circuit diagrams, PCB layouts, parts lists, sample software, and support documents, while actual device evaluation is possible using the optional LogiCoA001-EVK-001 evaluation board.
Going forward, ROHM will continue to develop LogiCoA MCUs to support various power supply topologies, contributing to achieving a sustainable society by making the power supply block (which accounts for the majority of power loss in applications) more energy-efficient and compact.
LogiCoA Brand
LogiCoA is a brand that embodies a design philosophy of fusing digital elements to maximize the performance of analog circuits. By combining the advantages of analog circuitry with those of digital control, it is possible to maximize the potential of circuit topologies, contributing to more efficient power utilization. As LogiCoA is a design concept that can be applied not only to the power supply field, but also to power solutions as a whole, ROHM is considering its application in future products and solutions.
Details of the LogiCoA Power Supply Reference Design
The REF66009 evaluation reference design offered on ROHM’s website allows users to verify the functionality of the LogiCoA MCU along with the basic operation of the LogiCoA power supply solution using a non-isolated 12V buck converter circuit. Sample software available on the reference design page makes it possible to confirm the sequence control of execution tasks and the monitoring of various parameters in the actual set using the LogiCoA001-EVK-001 reference board. For more information on the reference board, please contact a sales representative or the contact page on ROHM’s website.
Application Examples
- Industrial robots
- Semiconductor manufacturing equipment
- Gaming applications
Supports mounting in a wide range of general industrial equipment and consumer devices (30W to 1kW).
About the LogiCoA MCU
ROHM is developing LogiCoA MCUs optimized for integrated analog-digital control such as LogiCoA power supply solutions. Features include a built-in 3ch analog comparator that can be linked to a timer and D/A converter that enables digital control of various parameters to support different power supply topologies.
■ LogiCoA MCU Specifications (Tentative)
Availability: Now (LogiCoA MCU samples)
Terminology
Fully Digital Control Power Supply
A power supply controlled using digital technology. High-speed CPUs and DSPs can be used to precisely monitor and control various parameters such as voltage and current, improving power supply efficiency and reliability. What’s more, functions that are difficult to perform with analog control can be achieved, such as acquiring operation log data. However, CPUs and DSPs are expensive and consume a large amount of power, which can be a bottleneck in terms of costs and energy efficiency.
Analog Control Power Supply
A power supply configuration consisting of analog components. This type has become mainstream for power supplies 1kW and below due to its simplicity and low power consumption. On the other hand, implementing advanced functionality such as setting arbitrary parameters and logging data is difficult, requiring fully digital control that entails high costs and power consumption.
CPU (Central Processing Unit)
Responsible for executing programs and processing data. Handles calculations and processing as well as carrying out instructions according to a program.
DSP
A device that digitizes analog signals and performs operations such as analysis, filtering, and amplification on the converted digital signals. Flexible enough for high-speed processing and various applications, it plays an important role in circuits that handle digital signals, such as audio and image processing in addition to power supplies.
The post ROHM Offers LogiCoA: the Industry’s First* Analog-Digital Fusion Control Power Supply Solution appeared first on ELE Times.
Toshiba Demos New Switching Topology for Non-Isolated DC-DC Converters
Moved in 12 months ago, finally built a dedicated workbench for the first time
![]() | I’ve always had a makeshift workbench all my life, finally own a house with space that is just mine to do what I want with. [link] [comments] |
SMT vs. Through-Hole Assembly: Making the Right Choice for Your Project
The world of electronic manufacturing changes regularly, and as a result – fabricators must select one of two approaches in fabricating electronic components which are the Surface Mount Technology and Through-Hole Assembly methods. Each method brings its own set of advantages and drawbacks wherein you will have the final say on which manufacturing approach is geared to your standards and budget.
In this article, we’ll be taking a look at the nuances between SMT assembly and Through-Hole Assembly and the criteria that you’ll use as a basis to make the right decision on which manufacturing approach you’ll utilize for your project.
Surface Mount Technology (SMT): An Overview Definition of Surface Mount TechnologyTo begin our discussion, let’s learn what Surface Mount Technology is. SMT assembly is a process wherein electronic components are mounted directly onto a PCB’s surface through assembly machinery which an operator programs. As a result, this facilitates a fast-paced production which allows the creation of many surface-mounted circuit boards in a short timeframe.
Key Traits of SMT Assembly- Faster production setup – Compared to Through-Hole assembly, SMT assembly’s production setup facilitates fast-paced mass-production of printed circuit boards. This trait is what makes it favorable for factories or facilities that are required to mass-produce PCBs for different clients from different niches.
- High component density, small board size – Printed circuit boards that are made through this method of assembly are far smaller than their through-hole-made counterparts. That’s because the circuit board’s surfaces contain a very high density of electronic components which maximizes the use of the allotted space of the printed circuit boards
- Flexible application – Compared to through-hole boards, SMT-assembled PCBs are quite ubiquitous as they are utilized in most applications – usually in domestic devices or consumer goods. These items where SMT-assembled circuit boards are integrated into can range from kitchen appliances to your phone.
- Can facilitate more connections – Aside from their density, SMT-assembled boards are also known for their capability to accommodate more connections as components can be placed on both the front and back sides of the printed circuit board. As a result, there are more connections possible for every printed circuit board that works as intended.
- Requires specialized tools and expert-leveled operators – SMT-assembled PCBs will require more care in the process of manufacturing them due to the compact sizes of the printed circuits requiring more attention to ensure that the end product isn’t defective. To ensure that outcome, SMT assembly factories will be required to invest in specialized tools for manufacturing, assembly, and repairing, as well as hiring expert operators who will oversee the manufacturing process.
Before we delve into selecting the ideal assembly method for your project, let’s understand what
is through-hole assembly first. The through-hole method of assembling printed circuit boards involves drilling holes into the board’s surface. Once the holes are set, leads are inserted into the drilled holes before the components are installed and soldered on them. This method of assembly is significantly slower due to the added steps in installing the components but still has a key niche to fill in the world of electronics.
The Key Characteristics of Through-Hole Assembly
- Provides clients with specialized circuit boards – Despite the limited range of designs that through-hole assembled circuits have compared to SMT assembly-made circuit boards, it fares well in electronic setups that require a PCB that can function in specific conditions – say for example, a through-hole assembled PCB that is meant for use in electrical circuit setups that has a high heat dissipation for use in an industrial configuration.
- Reliable and resilient – Compared to the mass-produced SMT-assembled boards, through-hole assembled PCBs are more reliable due to the components being embedded onto the surface of the board through drilled holes and leads which also keeps the component intact. As a result, through-hole assembled boards have a very robust connection making the PCBs ideal for intense work environments ranging from military and aerospace applications to industrial use. On top of that, through-hole assembled boards sport better durability due to their designs having a high heat and stress tolerance.
- Easier repair time and adjustability – Unlike their SMT assembly counterparts, the PCBs made by through-hole assembly methods are easier to repair or operate manually. That’s because the key components embedded on the PCB are easier to replace or modify their positioning when necessary. In addition to what’s stated, the parts are easy to discern and identify so if a component on the PCB is defective; all it takes is to remove the defective part and replace it with a functioning version.
Now that we’ve covered the two methods of assembling circuit boards; let’s cover next the factors that you’ll need to consider in selecting which method best suits your project. Listed below are the factors that could play into choosing whether you will settle for SMT assembly or through-hole assembly for your future PCBs:
1. Costs of your ProjectThe first factor that comes into play in making the right choice for your PCB project is its cost. Are you willing to invest a lot of money for a specialized printed circuit board or will you settle for a PCB that can be applied in a myriad of situations? You should also determine how much is the total costs of the materials and components that will be used in your project.
These factors related to the project’s costs should help whether you should opt for SMT assembly or through-hole assembly.
2. Performance or QuantityThe next factor that comes into play whether you should choose SMT assembly or through-hole assembly is whether you value the quantity or the performance of the printed circuit board. If your goal is to produce as many printed circuit boards to meet the needs of many devices, then you could opt for SMT assembly.
However, if you value performance more – then you can consider aiming for through-hole assembly instead. Keep in mind though, that SMT-assembled circuit boards do still hold a solid performance which we’ll explore that nuance in the next criteria.
3. Choosing Between Flexibility or SpecializationIf you’ve factored in the performance of the PCBs for your project, then another layer of nuance comes into play – which is choosing between flexibility or specialization. If your project aims to turn the PCBs into a size-fits-many solution for different devices then you could opt for SMT assembly. As stated earlier, the best example of a flexible-use printed circuit board are domestic and everyday electronic items that we use such as smartphones, televisions, and the like.
However, if your electronic configuration calls for a specific circuit board; say for example – if your work environment favors a resilient PCB that can withstand intense amounts of workloads, mechanical stress, and electrical flow – you would want to settle with through-hole assembly instead of SMT-assembled boards as they could deteriorate fast in these types of working conditions. Notable examples of these intense work conditions where through-hole PCBs see use are automotive factories and industrial plants; aside from military and aerospace applications.
4. The Project’s Intended ApplicationThe last hurdle you’ll need to factor into your project whether you should settle for SMT assembly or through assembly is the intended application of your project. Is your project intended for use in consumer products or everyday items? If that’s the case then SMT assembled PCBs are your best bet.
However, if your project’s intended use is for specific niches or uses – for example; printed circuit boards for use in high-powered lighting, or to power the avionics inside a plane then utilizing through-hole assembled circuit boards could be the way to go.
Make the Right Decisions to Bring Your Project to RealityTo sum up what we’ve covered – there are two ways a printed circuit board is manufactured; namely through SMT assembly or through-hole assembly. To ensure that your project will work as intended for its target niche, you should consider the costs, quantity, or performance, its role whether it is flexible or specialized, and its intended niche.
Putting together the manufacturing methods and the factors that you need to consider will ensure that your electrical project is brought to reality.
The post SMT vs. Through-Hole Assembly: Making the Right Choice for Your Project appeared first on Electronics Lovers ~ Technology We Love.
Сторінки
